Does Prison Harden Inmates? - Study on Effects of Confinement, Chen Shapiro, 2005
Download original document:
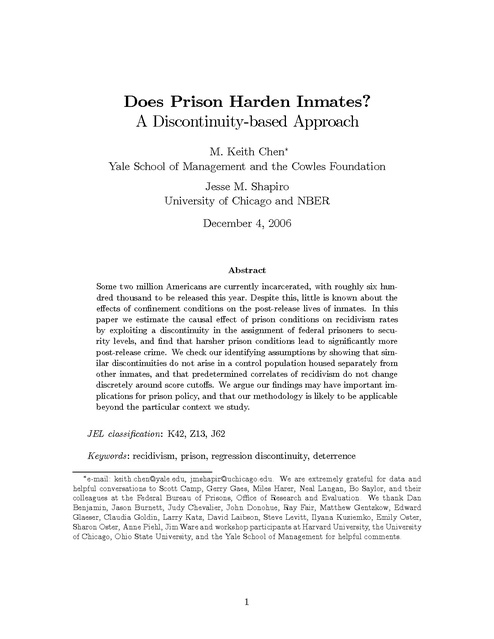
Document text
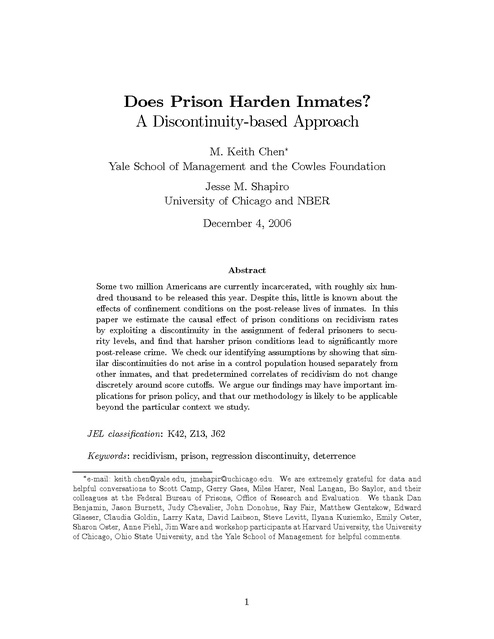
Document text
This text is machine-read, and may contain errors. Check the original document to verify accuracy.
Does Prison Harden Inmates? A Discontinuity-based Approach M. Keith Chen∗ Yale School of Management and the Cowles Foundation Jesse M. Shapiro University of Chicago and NBER December 4, 2006 Abstract Some two million Americans are currently incarcerated, with roughly six hundred thousand to be released this year. Despite this, little is known about the effects of confinement conditions on the post-release lives of inmates. In this paper we estimate the causal effect of prison conditions on recidivism rates by exploiting a discontinuity in the assignment of federal prisoners to security levels, and find that harsher prison conditions lead to significantly more post-release crime. We check our identifying assumptions by showing that similar discontinuities do not arise in a control population housed separately from other inmates, and that predetermined correlates of recidivism do not change discretely around score cutoffs. We argue our findings may have important implications for prison policy, and that our methodology is likely to be applicable beyond the particular context we study. JEL classification: K42, Z13, J62 Keywords: recidivism, prison, regression discontinuity, deterrence ∗ e-mail: keith.chen@yale.edu, jmshapir@uchicago.edu. We are extremely grateful for data and helpful conversations to Scott Camp, Gerry Gaes, Miles Harer, Neal Langan, Bo Saylor, and their colleagues at the Federal Bureau of Prisons, Office of Research and Evaluation. We thank Dan Benjamin, Jason Burnett, Judy Chevalier, John Donohue, Ray Fair, Matthew Gentzkow, Edward Glaeser, Claudia Goldin, Larry Katz, David Laibson, Steve Levitt, Ilyana Kuziemko, Emily Oster, Sharon Oster, Anne Piehl, Jim Ware and workshop participants at Harvard University, the University of Chicago, Ohio State University, and the Yale School of Management for helpful comments. 1 America’s jails and prisons house roughly two million inmates (Bureau of Justice Statistics, 2002), nearly twice as many as in 1990 and more in per capita terms than any other OECD country (OECD, 2001). Current and former prisoners constitute an increasingly large share of the U.S. population, yet little is known about the effects that imprisonment and prison conditions have on the subsequent lives of inmates.1 This omission is unfortunate: each year roughly six-hundred thousand people are released from incarceration (Bureau of Justice Statistics, 2002), and roughly two-thirds of those released will be rearrested within three years (Langan and Levin, 2002). Crimes by former inmates alone thus account for a substantial share of current and future crime. Moreover, unlike many determinants of crime, prison conditions are directly under the control of policymakers and the criminal justice system. Understanding the effect of confinement on post-release criminal activity is therefore essential to effective crime-control policy.2 Theory alone cannot tell us whether an increase in the severity of prison conditions will increase or decrease the propensity of inmates to commit crimes after release. Models of “specific deterrence” (Smith and Gartin, 1989), which posit that criminals learn from their own experiences about the severity of penalties, predict that harsher conditions will decrease the propensity to recidivate. Alternatively, if harsher prison conditions correspond to inferior labor market outcomes (as suggested by Western, Kling, and Weiman, 2001), or if prison life induces a taste for violence (Banister, Smith, Heskin and Bolston, 1973), then harsher conditions may lead to more crime following release. More generally, a growing literature on social interactions highlights the influence of peer effects on criminal behavior (Glaeser, Sacerdote and Scheinkman, 1996; Bayer, Pintoff, and Pozen, 2003). During incarceration, inmates may acquire skills, learn of new prospects, or develop criminal contacts. 1 A notable exception is Bayer, Pintoff, and Pozen (2003), which focuses on the effects of social interactions among juvenile on subsequent criminal behavior. Camp and Gaes (2003) study the effects of prison conditions on in-prison misconduct. 2 For example, the literature on prison privatization has recently focused much of its attention on whether private prisons are likely to provide lower quality services than publicly managed prisons (Hart, Shleifer, and Vishny 1997; Camp and Gaes, 2001). If prison conditions affect rates of post-release crime commission, then providing quality-based incentives to private prison managers becomes an even higher priority. 2 In this paper we exploit a feature of the federal inmate classification system to estimate the effect of moving a prisoner to a higher security level. Prior to incarceration, every federal inmate is assigned a score intended to reflect his need for supervision. An inmate is then assigned to a prison security level depending on where his score falls relative to certain predetermined cutoff values. By comparing inmates on either side of the boundaries between different security levels, we estimate the effect on recidivism of being assigned to a higher security level. Since both the physical and social conditions of confinement vary dramatically with security level, this setting provides a quasi-experiment for identifying the effect of prison conditions on post-release outcomes. Our approach avoids the obvious confounds inherent in simply comparing rearrest rates of prisoners in different security levels. Even with controls for demographics, such an estimation strategy would ignore the fact that prisoners are assigned to security levels based on characteristics such as crime severity that are themselves likely to predict recidivism. By taking careful account of the assignment mechanism, we can avoid bias introduced by the endogeneity of security level. We find that moving a prisoner over a cutoff that increases his assigned security level from minimum to low security roughly doubles his hazard rate of rearrest in a three-year follow-up window. We check our identifying assumptions by showing that similar discontinuities do not arise in a control population housed separately from other inmates, and that predetermined correlates of recidivism do not change discretely around score cutoffs. Although our conclusions are somewhat limited due to small sample sizes, in general they are difficult to reconcile with models in which “specific deterrence” or in-prison rehabilitation play a central role, and seem more consistent with models of social interactions or psychological effects of incarceration. This paper makes several contributions relative to the existing literature. Whereas most economic analyses of policy influences on crime focus on the deterrence or incentive effects of punishments (Levitt, 1998) or prison conditions (Katz, Levitt, and Shustorovich, 2003), we show that the conditions of incarceration may have a substantial influence on post-release criminal behavior. Our finding that harsher impris3 onment conditions cause greater recidivism stands in contrast to prior evidence of a specific deterrence effect (Sherman and Berk, 1984), in which punishing a criminal more severely reduces that individual’s subsequent probability of recidivism. Methodologically, the paper brings regression-discontinuity analysis (Campbell and Stanley, 1963; Rubin, 1977) to the study of post-release crime. In the prior work most closely related to our own, Berk and De Leeuw (1999) use a regressiondiscontinuity design to evaluate the impact of confinement conditions on in-prison misconduct, but not on post-release criminal activity. Moreover, although our research uses data from the federal corrections system, many state systems also employ scoring methods to assign inmates to confinement conditions. Our approach may therefore have wider applicability beyond the context we study.3 In addition, though we focus primarily on the impact of prison conditions on post-release crime, a similar methodology could be employed to study effects on labor-market attachment, family structure, and other post-release outcomes, all of which might respond significantly to the conditions of confinement. Our paper also contributes to a growing economic literature on the importance of peer effects in general (Sacerdote, 2001), and on the role of peer effects in criminal behavior in particular (Glaeser, Sacerdote, and Scheinkman, 1996). Unlike Bayer, Pintoff, and Pozen (2003), we do not attempt to directly measure the effects of changes in peer group composition on prisoner outcomes. However, the composition of a prisoner’s fellow inmates varies dramatically with the prisoner’s security level, and is therefore likely to form part of the effect of security level on post-release recidivism. Finally, our estimates suggest that the impact of prison conditions on recidivism is an important factor in designing effective prison systems. As we discuss in section 3, the effect of harsher conditions on recidivism must be weighed against their deterrent effect in order to determine whether more or less harsh conditions are optimal from a crime-control perspective. However, our point estimates are relatively large compared to existing estimates of deterrence effects, suggesting significant gains from 3 Indeed, since the first version of our paper was circulated, at least two studies have used discontinuity-based designs to evaluate the effects of incarceration (Pintoff, 2005) and sentence length (Kuziemko, 2006) on recidivism. 4 incorporating recidivism effects into policy analysis. The remainder of the paper is organized as follows. Section 1 discusses the relationship between security level and conditions of confinement and describes the dataset. Section 2 presents our findings as well as some checks on the plausibility of our identifying assumptions. Section 3 discusses policy implications of our findings. Section 4 concludes. 1 Background and Data Description 1.1 Inmate Classification and Security Level Upon entry to the federal prison system, an inmate is processed using an Inmate Load and Security Designation Form (see Figure 1). The Security Designation Data recorded on this form are used to produce the individual’s security custody score.4 In the construction of this score, each of seven items contributes points to an overall sum. For example, offenses are grouped into five categories, from lowest severity (such as “counterfeiting, under $2000”) to greatest severity (such as homicide), and each inmate receives an associated offense severity score ranging from 0 (least severe) to 7 (most severe). The scoring is done by a Regional Designator at the Bureau of Prisons, and follows a procedure laid out in detail in the Bureau of Prisons Security Designation and Custody Classification Manual (Federal Bureau of Prisons, 1982). Important for our identifying assumption is that no aspect of the score requires the Designator to exercise any personal judgment; all crimes, sentences, and judicial recommendations translate directly into a unique scoring. In the Appendix we discuss in detail how the components of the score are determined, and Appendix Table 1 summarizes how those components sum to the overall score. Once the score has been computed, it is compared to a set of cutoff values (see Appendix Table 2) to determine an inmate’s security level. Once a security level has 4 The score is intended to predict prisoner misconduct and therefore to measure the supervision needs of individuals. Over time, the score has been refined through continuing research into the predictors of prisoner misconduct (Harer and Langan, 2001). 5 been assigned to an inmate, a BOP employee assigns the inmate to an initial facility based primarily on location and on the availability of space.5 In some cases security level can change during the incarceration period at the discretion of a Bureau of Prisons (BOP) official, for example because of inmate misconduct. As such changes are endogenous, we will focus on security level upon entry to the federal prison system. Some considerations may intervene to break the link between score and security level. For example, deportable aliens may not be housed in minimum security, nor can those who have been convicted of threats to government officials.6 Such issues are recorded on the security designation form as public safety factors, and most have the effect of excluding an inmate from minimum security. Note, however, that our identification strategy does not use the variation in security level created by these exceptions. Rather, we will identify the effects of security level using the discontinuities in the relationship between score and recidivism that occur at the cutoff values. An inmate’s assigned security level has an enormous impact on his experiences in prison. As Appendix Table 1 details, prisoners convicted of more severe offenses, prisoners with more serious prior records, and prisoners with histories of violence are all, by design, more likely to be placed in more secure facilities. Thus comparing prisoners in different security levels one would find that those housed in more secure facilities are exposed to more violent individuals with more serious criminal histories. Given the growing literature on peer effects and the intensity of contact co-housed prisoners experience, this alone would suggest large security-level effects on postprison characteristics. Very few anthropological or ethnographic studies compare facilities with different security levels.7 Moreover, the dataset we use in this paper does not contain much detail about the in-prison experiences of the inmates. However, a different dataset, the Survey of Inmates of Federal Correctional Facilities (U.S. Department of Justice, 5 Inmates who suffer from chronic medical conditions are also assigned scores, but are housed separately in a prison medical facility. We will use this subsample as a control group to check the plausibility of our identifying assumptions. 6 Other such considerations include medical and mental health, aggressive sexual behavior, offense severity, organized crime, and gang membership. 7 Accounts of life in prison typically focus on one institution, usually maximum security (Sykes, 1958; Conover, 2001). 6 1991) contains data on inmate demographics, criminal histories, experiences in prison, and self-reported conditions of confinement for a nationally representative sample of federal inmates.8 Table 1 presents some simple comparisons across security levels, both in selfreported conditions of confinement and in-prison misconduct. The data strongly confirm the intuition that more secure facilities allow less contact with the community and less freedom of movement. While 14% of minimum security inmates report having been allowed furloughs during their current period of confinement, only 2.5% of low security inmates have had furloughs; for maximum security inmates the figure is below 1%. Similar trends show up in the percent of respondents who have been seriously injured during confinement. Moving from minimum to low security exposes an additional 2.7% to serious injury; moving from low to medium or medium to maximum increases the rate of injury by 1.2 and 1.8 percentage points, respectively. On the whole then, the available evidence strongly suggests that conditions of imprisonment differ dramatically by security level. Higher security prisons involve less contact with the outside world, allow less freedom, and subject inmates to far more violence. 1.2 Data Our data are a representative sample of 1,205 inmates released from federal prisons in the first six months of 1987 (Harer, 1994). Data on demographic characteristics and criminal histories were recorded for all inmates in the sample, as were the inmates’ security custody scores and security levels on entry to the system, when available.9 Following release, the FBI provided records of all re-arrests on either state for federal charges within a three year window of release. Hence even though all inmates in our 8 While using self-reported data to compare conditions across security levels does raise some methodological issues, Camp (1999) has found that such surveys do contain information helpful in making comparisons between facilities. 9 In many cases–usually inmates who entered the system prior to the introduction of modern computer records–data from the initial classification form was not available. In these cases score and security level were recorded from the earliest available reclassification form. The components of the score are unlikely to change during confinement, and conditional on time of entry, we find that our conclusions are quite similar (and statistically indistinguishable) across the two groups. 7 sample were initially incarcerated for federal crimes, we have records of all subsequent re-arrests within 3 years, even if they took place under state jurisdiction. Of the original sample of 1,205 inmates, security level data are missing for 16, and 11 served short sentences in halfway houses that do not have a security designation. Another 216 were placed in administrative facilities for special medical needs; we will later use this sub-sample as a control group in our analysis. Finally, 12 inmates have missing data on score and 2 have miscoded rearrest dates (with rearrest occurring prior to release), leaving a total sample of 948 with usable data. Table 2 presents summary statistics for this group. Over half of all of inmates were rearrested within three years of release, a level comparable to most state-level studies of recidivism (Camp and Camp, 1997). Other sample characteristics are less surprising: relative to the U.S. population, the sample contains more males, fewer whites, fewer high school graduates, and more previously convicted offenders. Grouping by security level, Table 2 also demonstrates the large changes in these characteristics across levels. For example, the percent of convicts rearrested within 3 years is 38% in minimum security, but jumps to 55% for low security, and is 60% for all levels higher then low. In these level statistics the most dramatic changes occur when leaving minimum security, leading us to suspect that our strongest results will come from this break. A crucial requirement for our analysis is that security level vary discontinuously with score. As Figure 2 demonstrates, the data confirm what policy implies: the probability of being placed in low rather than minimum security jumps discretely when the score passes the official cutoff of 6. Similar jumps are visible at each cutoff (see Appendix Table 3). 2 Results Given how drastically prison conditions vary across security levels, it is plausible that the type of an inmate’s prison greatly affects his post-prison outcomes. To test this we exploit the fact that the assignment process outlined in Section 1 exhibits dis8 continuities at several pre-determined cut-off points. Inmates who find themselves at opposite ends of any of these cutoffs are likely to be ex-ante comparable in all underlying attributes, providing us with a quasi-experimental way of testing the effects of security level. 2.1 Regression Discontinuity In a regression-discontinuity design (Campbell and Stanley, 1963; Rubin, 1977; Berk and De Leeuw, 1999), subjects are assigned a treatment condition based on cutoff values of a known and measured assignment score. For federal inmates the security designation score discussed in Section 1.1 serves this purpose.10 By conditioning our analysis of recidivism on both an inmate’s score (constrained to enter smoothly) and his resulting security level, we can obtain consistent estimates of the treatment effect as long as pre-determined inmate characteristics vary smoothly with the security custody score. In essence then, we argue that within a small interval around a cutoff the allocation of prisoners to different security levels amounts to a random assignment procedure. Note that, because we will identify the effects of security level assignment on recidivism using only variation induced by the cutoffs, our procedure does not require us to assume that all determinants of recidivism propensity are well proxied by the security designation score, or that the score is the sole determinant of inmate placement. Rather, it requires only that unmeasured determinants of recidivism do not vary discontinuously with the assignment score. As a first pass at the data, Figure 3 presents one-year rearrest probabilities for each security custody score from 0 through 9.11 The figure also presents the average probability of rearrest after one year as predicted by a probit model using prede10 Regression discontinuity is not new to the study of crime. Berk and Rauma (1983) investigate the effects of transitional aid to prisoners on recidivism, exploiting a California policy which extends unemployment insurance to prisoners who work a certain number of hours prior to release. Berk and de Leeuw (1999) also study the California prison system, using a regression discontinuity design to predict the effects of various assignment procedures on in-prison misconduct. In other contexts, economists have use regression discontinuity to estimate the effects of financial aid on college enrollment (van der Klaauw 2001), the effect of incumbency on election results (Lee, 2001), and the effects of class size on school performance (Hoxby, 2000). 11 See Appendix Table 3 for rearrest percentages for a wider range of scores. 9 termined inmate characteristics (age, education, race, gender, and employment and marital status) as independent variables. As the figure shows, the relationship between actual recidivism and security custody score closely resembles the relationship between predicted recidivism and score, except around the change between score 6 and score 7. As noted above, inmates with a score of 7 are typically assigned to low security prisons, whereas inmates with a score of 6 are usually placed in minimum. The fact that actual recidivism, but not recidivism predicted based on predetermined characteristics, jumps up at this point is the basis for our approach to estimating the effect of security level on subsequent criminal behavior. The figure also illustrates a limitation of our context relative to other areas in which regression discontinuity designs have been employed: since security custody scores take on only a small number of integer values, there is a limit to how close to the cutoffs we can look. We will attempt to alleviate this concern by testing formally for the presence of discontinuities in observed and unobserved inmate characteristics around the cutoff values. In the analysis to follow, we will test formally for a discontinuity at the cutoff point. We will also show evidence that predetermined correlates of recidivism do not change discontinuously around the cutoff, suggesting that the discontinuity in recidivism rates is not a result of unobserved inmate heterogeneity. Although small samples make our data noisier than would be ideal for such an analysis, we will argue that the evidence as a whole points to a significant causal impact of security level placement on post-release recidivism. 2.2 Reduced-form Estimates of the Effect of Score Cutoffs To analyze the data more formally, we will estimate both a Cox proportional hazard model of rearrest rates and a probit analysis using as dependent variables the probability of being rearrested after 1, 2, or 3 years following release. Our independent variables will be polynomial terms in score, demographic controls, and dummies for the three score cutoffs relevant to our data. Since having a score above a certain cutoff does not guarantee placement in a higher security level (see Figure 2 and Appendix Table 3), the results in this section will be reduced-form estimates of the effect of 10 score cutoffs on recidivism. In subsection 2.4 we will present estimates that can be interpreted more directly as the effect of security level on rearrest. The Cox proportional hazard model assumes that an underlying hazard rate of failure is multiplicatively shifted by changes in right-hand-side variables. In our study the survival time is the time until the prisoner is rearrested, with individuals not rearrested during the follow-up period treated as censored observations. The advantage of the proportional hazard assumption is that it does not require functional form assumptions about the baseline hazard rate of recidivism. The model we estimate will treat the hazard rate of rearrest h(t) as given by h(t) = h0 (t) exp (λg(score) + α1 S6 + α2 S9 + α3 S13 ) (1) where h0 (t) is the baseline hazard function, g(score) is a fourth order polynomial in the security custody score,12 and Sn are dummies for score > n. The parameters αn capture the effects of score cutoffs on the hazard rate of rearrest. The assumption required to identify the causal effect of the score cutoffs Sn is that all omitted characteristics vary continuously with score. Column (1) of Table 3 presents our estimates of the proportional hazard model. The coefficients reported are model parameters, and can be exponentiated to obtain hazard ratios. The first coefficient implies a statistically significant positive effect of the first security custody score cutoff on the probability of rearrest, equivalent to an approximate doubling of the underlying hazard rate. Even at the lowest end of our confidence interval, exceeding the first score cutoff raises the hazard rate of rearrest by five percent. While our estimates are not very precise, we reject the hypothesis that harsher conditions lead to reduced recidivism, suggesting that “specific deterrence” or rehabilitation are not the dominant forces in this context. Indeed, our point estimates indicate positive effects on recidivism at higher scores, although our standard errors do not allow us to reject the null effect for higher cutoffs. Because of this imprecision we focus primarily on the first cutoff, between scores 6 and 7. 12 We chose a fourth-order polynomial by iteratively adding polynomial terms until the last term is not statistically significant. Results are similar using a third- or fifth-order polynomial. 11 The proportional hazard model allows us to avoid making assumptions about the shape of the hazard function over time, but this may also mask interesting heterogeneity in the treatment effect at different time horizons. We therefore supplement the proportional hazard model with a series of probit models that treat recidivism in one-, two-, and three-year follow-up windows as dependent variables. In particular, we estimate models of the form: P (Rt ) = Φ(βX + λg(score) + α1 S6 + α2 S9 + α3 S13 ) (2) where Rt is 1 if an inmate has recidivated after t years and 0 if he has not and Φ is the normal CDF. Columns (2) through (4) of Table 3 present the results of this analysis. Controlling for a polynomial in security custody score, there is in general a positive effect of prison security level on the probability of post-release rearrest. The largest effect of the first score cutoff occurs in a two-year follow-up window, where placement above a score of 6 leads to a 25 percentage point increase in the probability of rearrest. This effect is statistically significant at the five percent level, whereas the effects at one- and three-year horizons are weaker and only marginally statistically significant. Again, despite our lack of precision, we find fairly consistent evidence for a positive causal link between harsh prison conditions and recidivism, and we can definitively reject negative effects of harsher conditions with any appreciable magnitude. 2.3 Robustness and Specification Checks The estimates we have presented require that all correlates of recidivism vary continuously with the security custody score. While it is not possible to test this assumption for all covariates, we can ask whether observed covariates meet this criterion. Table 4 tests for discontinuities in our control variables, estimating probit models that parallel equation (2) but with demographic characteristics (high school degree status, prior convictions, marital status, race, and employment) recorded on entry to prison as dependent variables. Only one demographic characteristic has a marginally significant 12 (at the 10 percent level) discontinuity at a score cutoff; the rest show no significant changes. Thus in general we do not observe significant changes in subject characteristics around our identifying cutoffs. We should note, however, that the confidence intervals in these models are wide, giving these tests limited power. Panel A of Table 5 shows estimates of the models from Table 3 with controls for the demographic variables used in Table 4. We focus on the effect of the first score cutoff since this is where we have the most data. Column (1) of Panel A shows that including demographic controls reduce our estimated effect of exceeding the first score cutoff from a doubling of the relative hazard of rearrest to a 50 percent increase in rearrest probability. The estimated coefficient in the model with controls is still large, but we can no longer reject the null hypothesis of no effect. In the probit models reported in Columns (2) and (3) of Panel A, we also see modest decreases in the point estimates, although in this case the estimates do remain marginally statistically significant. Finally, in Column (4) we show that including demographic controls substantially reduces the estimated effect of score cutoff on three-year recidivism rates, making this estimate statistically indistinguishable from zero. On the whole, while we are not able to consistently reject the null hypothesis, the specifications with demographic controls do still suggest a positive effect of harsh conditions on rearrest probabilities. Thus far we have only considered whether our results are driven by observed heterogeneity among inmates. An alternative approach that can also capture the effects of unmeasured heterogeneity is to examine a population with known scores that is not housed in accordance with the security guidelines of those scores. Inmates housed in “administrative” facilities, which are essentially prison hospitals, constitute just such a population. They are housed apart from the general population and are therefore not exposed to the variation in conditions of confinement that we discussed in Section 1. Our dataset contains 211 inmates with known scores who were initially assigned to administrative facilities. Overall these inmates exhibit similar rates of recidivism to the general inmate population, and we find that similar demographic characteristics predict recidivism in both groups. In Panel B of Table 5, we test for a discontinuity among this group as a “placebo” exercise: if unobserved heterogeneity 13 among inmates were driving our results, then we would be likely to estimate similar discontinuities for this administrative population. As Panel B of Table 5 reports, there is no evidence of a discontinuous relationship between score and recidivism for these inmates. Moving an inmate housed in an administrative facility from minimum to low security designation in general has an insignificant negative effect on the probability of rearrest. Small sample sizes mean that these results are imprecise and should be interpreted with caution; nevertheless, in one case (two-year rearrest rates) we can reject the equality of the coefficients for administrative and non-administrative inmates at the 10 percent level.13 While not conclusive, these findings are at least consistent with the hypothesis that the discontinuity observed among non-administrative inmates is a results of confinement conditions and not unobserved heterogeneity that changes discretely around score cutoffs. Another potential concern with our estimates is that our sample is representative of the released population, not the incarcerated population. Although the released population is of greater interest for many policy questions, it is important to know whether the effects we identify are weaker for the average inmate than for the average released inmate. In effect, this amounts to asking how our effects depend on sentence length, since those with longer sentences are less likely to be released at any given point in time, and are therefore less likely to enter our sample. Panel C of Table 5 shows the robustness of our results to weighting our estimates by total time in prison, and thus assigning more importance in the model to inmates who had relatively low probabilities of entering our sample. In general, the estimated effect of score cutoff becomes larger and statistically stronger. This suggests that our effects are not limited to inmates with short prison stays, and if anything may be more important for those who are incarcerated for longer periods. As Lee and Card (2006) have pointed out, regression discontinuity estimates suffer from specification error because polynomials can only approximate the smooth 13 In the sample of inmates housed in administrative facilities, there are 17 and 19 inmates at scores 6 and 7, respectively, suggesting a modest, but not tiny, amount of data available to test for a discontinuity at this point. 14 functions they are meant to capture. As they note, this type of error can induce score-level correlation in the error structure that can be addressed using a clustered standard error. Experiments with specifications using standard errors clustered by security designation score (not shown) reveal slight decreases in our standard errors; to be conservative we therefore present estimates with unclustered errors. A final concern is that our estimates measure the post-prison arrest rate, not necessarily the crime-commission rate. The claim that harsher prison conditions increase the commission of crimes rests on the assumption that the probability of arresting an ex-convict conditional on his having committed a crime does not depend on his former security level. For example, if upon release a low security inmate is subject to more frequent drug tests than his minimum security counterpart, our results may be picking up an increased probability of rearrest that has nothing to do with increased criminal tendencies. Indeed, Petersilia and Turner (1993) found using a randomized evaluation that more intensive post-release supervision increase rates of reincarceration by about 8 percentage points (see also Piehl and LoBuglio, forthcoming). While we cannot entirely rule out this explanation, we know of no federal parole policy that specifies a relationship between supervision intensity and security level of releasing facility, and we note that even the large differences in supervision intensity studied by Petersilia and Turner (1993) did not produce large enough effects to explain the majority of the effect we estimate.14 Finally, the effect of security level on recidivism is visible even if we exclude parole violations from our sample. Thus, while we cannot completely rule out a bias, it seems unlikely to account for most of our findings. 14 As additional data on this point, we note that most state parole agencies use standardized risk assessment tools to map inmates into supervision levels (Jones et al, 1999). None of the instruments we examined take account of an inmate’s former security level, nor look as if their cutoffs coincide with those in the security custody score. Furthermore, the variables these systems do take into account relate primarily to providing the appropriate services (drug users receive drug counselling) and limiting especially newsworthy crimes (convicted sex offenders are monitored very closely). 15 2.4 Structural Estimates The results in the previous section show the effect of exceeding score cutoffs on the probability of rearrest. Because the score cutoffs do not perfectly determine the security level in which an inmate is housed, the coefficients in Table 3 cannot be interpreted as estimates of the effect of security level on the probability of rearrest. To get such an estimate, we need to adjust the coefficients to correct for the imperfect link between security custody score and security level. We implement this estimation with a probit model of the following form: P (Rt ) = Φ (λg(score) + γ 1 (low) + γ 2 (low/medium) + γ 3 (medium)) (3) where low, low/medium, and medium are dummies for each respective security level category. Since these dummies are endogenous regressors, we model them as linear functions of the score cutoffs S6 , S9 , and S13 , which we have argued are exogenous conditional on the score polynomial g (score). Because we have not been able to obtain reliably convergent maximum likelihood estimates of this model, we will present estimates obtained using the procedure proposed by Newey (1987). (We note that both point estimates and statistical significance are similar if we use a linear probability model estimated using two-stage least squares.) Table 6 presents our estimates of the two-stage probit models with endogenous regressors. In general these estimates are too imprecise to rule out effects of zero, although the effect of a move from minimum to low security on two-year recidivism rates is significant at the 10 percent level. The point estimates consistently show sizable effects (on the order of about 30 percentage points) of moving an inmate from minimum to low security, which is where our data permit the best identification. 3 Policy Implications Our estimates suggest that harsher prison conditions induce substantially greater post-release recidivism among former federal inmates, an effect that would likely have important implications for prison policy. For several reasons however, our findings by 16 themselves do not imply that prison conditions should be less harsh or restrictive. In this section, we discuss additional considerations that must be weighed against our evidence in determining the net effect on crime of harsher (or less harsh) confinement conditions. The first potential counterpoint to our findings is that harsher prisons may suppress crime by deterring crime among the non-incarcerated. Indeed, using the inprison mortality rate as an index of a state’s prison conditions, Katz, Levitt, and Shustorovich (2003; hereafter KLS) use annual data to show that harsher prison conditions have a sizable contemporaneous deterrent effect. For a coarse comparison of the magnitudes of their findings and our own, we note that according to the 1990 Census of State and Federal Adult Correctional Facilities (Bureau of Justice Statistics, 1990), federal facilities with a medium-security custody designation had prisoner death rates of 2.28 per 1, 000 prisoners, whereas those with a minimum-security designation had death rates of 0.66 per 1, 000. Unfortunately the census does not contain death rates for low-security prisoners, which would be most relevant to our discontinuity between minimum and low-security prisons. However, while the difference in death rates between minimum and medium security facilities are likely to overstate the difference between minimum and low, in other surveys we find that the difference in rates of serious injury between minimum- and low-security facilities is almost as large as that between minimum- and medium-security facilities (see Table 1). This suggests that death rates in medium- and minimum-security facilities may reasonably approximate the differences faced by inmates on opposite sides of the cutoff we study. Given this approximation, KLS’s baseline estimates imply that moving all inmates from minimum- to low-security facilities would decrease the murder rate by 0.02 (per 100, 000 people), the violent crime rate by 10, and the property crime rate by 23, for a total reduction in crime of about 33. Note, however, that because KLS primarily focus on the contemporaneous effects of changes in in-prison mortality, their estimates are likely to omit the effect of harsher confinement conditions on future recidivism. Our estimates imply that every released inmate would be 28 percentage points more likely to commit a crime in the year following release if inmates were all housed in low 17 rather than minimum security facilities. Given that approximately 600, 000 inmates are released annually (accounting for about 200 of every 100, 000 Americans), even if each released inmate were to commit at most one crime, and that all crimes result in arrests (both conservative assumptions), our estimates would imply an increase in the crime rate of approximately 56 per 100, 000 released prisoners, for a net increase of about 23 crimes per 100, 000. In other words, the effects we estimate are on the same order of magnitude as the deterrent effects estimated by KLS; indeed they appear large enough to outweigh deterrence and drive a net increase in crime should prison conditions worsen. (An important caveat to this conclusion is that KLS find that lagged changes in the in-prison death rate continue to have negative effects on crime. If the recidivism effect of harsher prisons exceeded the deterrent effect we would have expected lagged effects to eventually become positive, which KLS do not find.) A second critical interpretational issue concerns the role of social interactions and peer effects. If the effects we estimate are primarily a result of confinement conditions per se, then it is reasonable to treat our estimates as measuring the potential consequences of changes in conditions on recidivism. If, on the other hand, the effects we compute result largely from prisoners’ effects on one another, then, to a first approximation, moving inmates from minimum to low security would have no effect on overall recidivism rates, because the inmates in low security would experience a reduction in recidivism rates from the inclusion of a minimum-security inmate in their peer group. Note, however, that if peer effects are nonlinear, or if the magnitude of peer effects depends on individuals’ ex-ante characteristics, then it may be possible to sort inmates so as to reduce overall levels of recidivism in the post-release population. A final issue regarding the consequences of changing prison conditions is that the security level of a facility is tailored to the inmate population in part to minimize the risk of in-prison misconduct, escape, and other undesirable outcomes. Indeed, Berk and de Leeuw (1999) show, using a discontinuity design, that inmates placed in higher security levels engage in less in-prison misconduct. Of course, these reductions in misconduct require the application of greater resources (such as staff). Nevertheless, if harsher conditions reduce in-prison violence and other misconduct, these effects must 18 be made a part of any complete analysis of policy regarding confinement conditions. 4 Conclusion With over two million inmates currently incarcerated and six hundred thousand inmates released per year, the demographic impact of American prisons can hardly be overstated. In this paper we have attempted to understand the impact of incarceration on inmates’ subsequent behavior, focusing on perhaps the most serious and socially costly consequence of that incarceration, recidivism into crime. By exploiting discontinuities in the assignment of inmates to different security levels, we attempt to isolate the causal impact of prison conditions on recidivism. Our findings suggest that harsher prison conditions cause higher rates of post-release criminal behavior, behavior which is also measurably more violent. We attempt to validate our discontinuity approach by showing that besides prison conditions, other observable characteristics do not jump at prison assignment cutoffs, nor do our jumps in recidivism appear in a control population housed in administrative facilities. Turning to policy questions, while our estimates are imprecise they are large in magnitude and appear larger than benchmark estimates of deterrence effects. Our results also highlight the potential importance of research aimed at determining which aspects of incarceration increase or reduce recidivism. A richer understanding of the ways inmates respond to both harsher prison conditions and exposure to more violent peers would likely allow planners to suppress socially costly recidivism by adjusting conditions and redesigning assignment systems, both between and within prisons. Additionally, because many prison systems utilize score cutoffs for inmate placement, our work highlights an empirical methodology with potentially wide scope and policy relevance. 19 References [1] P. A. Banister, F.V. Smith, K. J. Heskin, and N. Bolton. Psychological correlates of long-term imprisonment. British Journal of Criminology, 13(4):312—330, October 1973. [2] Patrick Bayer, Randi Pintoff, and David E. Pozen. Building criminal capital behind bars: Social learning in juvenile corrections. Yale University Mimeo, October 2003. [3] Richard A. Berk and J. de Leeuw. Evaluation of California’s inmate classification system using a generalized discontinuity design. Journal of the American Statistical Association, 94(448):1045—1052, 1999. [4] Richard A. Berk and David Rauma. Capitalizing on nonrandom assignment to treatments: A regression-discontinuity evaluation of a crime control program. Journal of the American Statistical Association, 78(381):21—27, March 1983. [5] Camille Graham Camp and George M. Camp. The Corrections Yearbook: 1997. Criminal Justice Institute, Inc., South Salem, New York, 1997. [6] Scott D. Camp. Do inmate survey data reflect prison conditions? using surveys to assess prison conditions of confinement. The Prison Journal, 79(2):250—268, June 1999. [7] Scott D. Camp and Gerald G. Gaes. Private prisons: What do we really know and why don’t we know more? In David Schichor and Michael Gilbert, editors, Privatization of Criminal Justice: Past, Present and Future, pages 283—298. Anderson, Cincinnati, OH, 2001. [8] Scott D. Camp and Gerald G. Gaes. Are prisons criminogenic? some experimental evidence. Unpublished manuscript, September 2003. [9] D. T. Campbell and J. C. Stanley. Experimental and quasi-experimental designs for research. Rand McNally, Chicago, 1963. [10] Ted Conover. Newjack: Guarding Sing Sing. Vintage Books, New York, NY, 2001. [11] Organization for Economic Co-operation and Development. Society at a Glance: OECD Social Indicators. OECD, Paris, 2001. [12] Edward L. Glaeser, Bruce Sacerdote, and José A. Scheinkman. Crime and social interactions. Quarterly Journal of Economics, 111(2):507—548, May 1996. [13] Miles D. Harer. Recidivism among federal prisoners released in 1987. 1994. [14] Miles D. Harer and Neal P. Langan. Gender differences in predictors of prison violent: Assessing the predictive validity of a risk classification system. Crime and Delinquency, 47(4):513—536, October 2001. 20 [15] Oliver Hart, Andrei Shleifer, and Robert Vishny. The proper scope of government: Theory and an application to prisons. Quarterly Journal of Economics, 112(4):1127—1161, November 1997. [16] Caroline M. Hoxby. The effects of class size on student achievement: New evidence from population variation. Quarterly Journal of Economics, 115(4), 2000. [17] Dana A. Jones, Shelley Johnson, Edward Latessa, and Lawrence F. Travis. Case classification in community corrections: Preliminary findings from a national survey. Topics in community corrections, 1999. [18] Lawrence Katz, Stephen D. Levitt, and Ellen Shustorovich. Prison conditions, capital punishment, and deterrence. American Law and Economic Review, 5(2):318—343, 1996. [19] Ilyana Kuziemko. Discretion versus rules in parole decisions: An empirical investigation. Harvard University Mimeograph, September 2006. [20] Patrick A. Langan and David J. Levin. Recidivism of prisoners released in 1994. Bureau of Justice Statistics Special Report, June 2002. [21] David S. Lee. The electoral advantage to incumbency and voters’ valuation of politicians’ experience: A regression discontinuity analysis of elections to the u.s. house. National Bureau of Economic Research Working Paper, 8441, 2001. [22] David S. Lee and David Card. Regression discontinuity inference with specification error. UC Berkeley Mimeograph, February 2006. [23] Stephen D. Levitt. Juvenile crime and punishment. Journal of Political Economy, 106(6):1156—1185, December 1998. [24] Whitney K. Newey. Efficient estimation of limited dependent variable models with endogenous explanatory variables. Journal of Econometrics, 36(3):231—250, November 1987. [25] Federal Bureau of Prisons. Security Designation and Custody Classification Manual. U.S. Department of Justice, Washington, D.C., October 1982. [26] Federal Bureau of Prisons. Security Designation and Custody Classification Manul. U.S. Department of Justice, Washington, D.C., 1985. [27] Joan Petersilia and Susan Turner. Intensive probation and parole. Crime and Justice, 17:281—335, 1993. [28] Anne Morrison Piehl and Stefan F. LoBuglio. Does supervision matter? In Jeremy Travis and Christy Visher, editors, Prisoner reentry and public safety in America. Cambridge University Press, Cambridge, UK, 2005. forthcoming. [29] Randi Pintoff. Juvenile jails: A path to the straight and narrow or hardened criminality? University of Maryland Mimeograph, November 2005. 21 [30] Donald B. Rubin. Assignment to treatment group on the basis of a covariate. Journal of Educational Statistics, 2:34—58, 1977. [31] Bruce Sacerdote. Crime and social interactions. Quarterly Journal of Economics, 116(2):681—704, May 2001. [32] Lawrence W. Sherman and Richard A. Berk. The specific deterrent effects of arrest for domestic assault. American Sociological Review, 49(2):261—272, April 1984. [33] Douglas A. Smith and Patrick R. Gartin. Specifying specific deterrence: The influence of arrest on future criminal activity. American Sociological Review, 54(1):94—106, February 1989. [34] Gresham M. Sykes. The Society of Captives: A Study of A Maximum Security Prison. Princeton University Press, Princeton, NJ, 1958. [35] Bureau of Justice Statistics U.S. Department of Justice. Census of State and Federal Adult Correctional Facilities. Inter-University Consortium for Political and Social Research, Ann Arbor, MI, 1990. [36] Bureau of Justice Statistics U.S. Department of Justice. Key facts at a glance. http://www.ojp.usdoj.gov/bjs/keytabs.htm, 2002. [37] Bureau of Prisons U.S. Department of Justice. Survey of Inmates of Federal Correctional Facilities, 1991. Inter-University Consortium for Political and Social Research, Ann Arbor, MI, 1994. [38] Wilbert van der Klauuw. Estimating the effect of financial aid offers on college enrollment: A regression-discontinuity approach. 2001. Forthcoming. [39] Bruce Western, Jeffrey R. Kling, and David F. Weiman. The labor market consequences of incarceration. Crime and Delinquency, 47(3):410—427, July 2001. 22 Table 1 Security Level and Prison Conditions Percent of Inmates Minimum Receiving a furlough Security Level Low Medium Maximum 14.20% 2.50% 1.60% 0.78% In cell for > 8 hours per day 49.01 55.21 55.03 58.22 Seriously injured 16.54 19.21 20.45 22.19 Found guilty of prison rule violation for: Possession of drugs 0.45 2.02 3.59 15.78 Possession of alcohol 0.11 0.47 2.63 9.53 Possession of a weapon 0.00 0.12 0.99 7.66 Assaulting an inmate 1.07 3.32 5.05 9.38 Assaulting a correction officer 0.00 0.36 1.04 5.94 1782 843 2315 640 Number of observations Source: Authors’ calculations based on U.S. Department of Justice (1991). Notes: In all cases, a Pearson χ2 test rejects the null hypothesis of equal proportions across security levels at the one percent level. 23 Table 2 Summary Statistics Security level Share rearrested within One year All Minimum 0.1624 0.1179 0.2195 0.2171 Two years 0.2732 0.1996 0.3659 0.3643 Three years 0.3681 0.2966 0.4329 0.4729 36.62 37.14 35.88 High school graduates 0.5591 0.6464 0.4634 0.4419 Previously convicted 0.6867 0.5837 0.8049 0.8217 Married as of arrest 0.3850 0.4354 0.3659 0.2946 Employed before arrest 0.5380 0.6369 0.4451 0.3953 White 0.7131 0.7643 0.6829 0.6279 Male 0.9219 0.8612 1.000 0.9961 948 526 164 258 Mean age Low >Low 36.02 Share of inmates who are: Number of observations 24 Table 3 Reduced-form Estimates of the Effect of Score Cutoffs on Rearrest Model (1) Cox (2) Probit (3) Probit (4) Probit Dependent Time to Probability of rearrest within variable rearrest One year Two years Three years Score>6 0.6999 (0.3338) 0.1826 (0.0976) 0.2515 (0.1105) 0.2003 (0.1155) Score>9 0.4611 (0.3359) 0.0424 (0.0857) 0.1627 (0.1217) 0.1702 (0.1337) Score>13 0.0460 (0.4942) -0.1022 (0.0510) 0.2353 (0.1960) 0.0365 (0.1950) Security custody 0.6852 score (0.1320) 0.1417 (0.0268) 0.1784 (0.0356) 0.1737 (0.0417) Score2 -0.1261 (0.0383) -0.0304 (0.0080) -0.0331 (0.0107) -0.0300 (0.0129) Score3 /100 0.7824 (0.3086) 0.2146 (0.0666) 0.1936 (0.0900) 0.1670 (0.1144) Score4 /10000 -1.5603 (0.7632) -0.4729 (0.1690) -0.3690 (0.2305) -0.2791 (0.3069) Observations 948 948 948 948 Pseudo-R2 – 0.1047 0.1224 0.1163 Notes: Standard errors in parentheses. In Cox model, reported coefficients represent underlying model parameters. In probit models, coefficients reflect marginal effects evaluated at the mean of the independent variables. 25 Table 4 Tests for Discontinuities in Predetermined Correlates of Rearrest (1) (2) (3) (4) Dependent variable is dummy for: High school Prior graduate convictions Married White (5) Employed before arrest Score>6 -0.0740 (0.1196) 0.1037 (0.1043) -0.2007 -0.0389 (0.1021) (0.1033) -0.1866 (0.1215) Score>9 -0.1229 (0.1384) -0.1958 (0.2035) -0.1683 -0.0855 (0.1245) (0.1189) -0.2100 (0.1512) Score>13 0.2454 (0.1508) -0.5038 (0.3187) -0.1947 -0.0404 (0.1601) (0.1746) -0.3230 (0.1799) Security custody score -0.1161 (0.0438) 0.1689 (0.0428) -0.0969 -0.1109 (0.0442) (0.0370) -0.1355 (0.0465) Score2 0.0127 (0.0138) -0.0368 (0.0148) 0.0134 0.0167 (0.0143) (0.0112) 0.0066 (0.0150) Score3 /100 -0.0414 (0.1229) 0.3300 (0.1540) -0.0329 -0.0906 (0.1331) (0.0946) 0.0731 (0.1405) Score4 /10000 -0.0112 (0.3324) -0.8399 (0.4616) -0.0501 0.1589 (0.3732) (0.2424) -0.3689 (0.3966) Observations 948 948 948 948 948 Notes: Standard errors in parentheses. Coefficients reflect marginal effects evaluated at the mean. 26 Table 5 Robustness and Specification Checks Model Dependent variable (1) Cox Time to rearrest (2) (3) (4) Probit Probit Probit Probability of rearrest within One year Two years Three years Panel A: Demographic controls Baseline 0.6999 (0.3338) 0.1826 (0.0976) 0.2515 (0.1105) 0.2003 (0.1155) Baseline + controls 0.4366 (0.3459) 0.1427 (0.0909) 0.1958 (0.1102) 0.1237 (0.1189) Panel B: Comparison with Administrative sample Baseline 0.6999 (0.3338) 0.1826 (0.0976) 0.2515 (0.1105) 0.2003 (0.1155) Administrative sample -0.0658 (0.4706) -0.0436 (0.1178) -0.1560 (0.1775) -0.0514 (0.2005) Z-test of difference 1.21 1.39 1.90 in coefficients (p = 0.225) (p = 0.164) (p = 0.058) 1.06 (p = 0.288) Panel C: Weights to reflect release probability Baseline (unweighted) – 0.1826 (0.0976) 0.2515 (0.1105) 0.2003 (0.1155) Weighted by time in prison – 0.2346 (0.0950) 0.2666 (0.1047) 0.3071 (0.1080) Notes: Standard errors in parentheses. Demographic controls include age and dummies for high school graduate, prior convictions, married, white, male, and employed prior to arrest. Coefficients in probit models reflect marginal effects evaluated at the sample mean of the independent variables. 27 Table 6 Structural Estimates of the Effect of Security Level on Rearrest (1) Dependent variable (2) (3) Probability of rearrest within One year Two years Three years Model coefficients: Low 1.1513 (0.8651) 1.7678 (1.0595) 0.9564 (0.8790) Low/Medium 1.7973 (1.2774) 2.8382 (1.5524) 2.0659 (1.2974) Medium 0.0983 (1.9798) 3.8255 (2.4139) 1.9682 (2.0395) Low-Minimum 0.2800 0.4634 0.3038 Low/Medium-Low 0.2450 0.3363 0.3566 Medium-Low/Medium -0.5089 0.2540 -0.0283 948 948 948 Average marginal effects: Observations Notes: Standard errors in parentheses. Models estimated using Newey’s (1987) procedure. Average marginal effects reflect change in average predicted probability of recidivism caused by indicated change in security level. 28 Figure 1 Inmate Load and Security Designation Form 29 Figure 2 Security Custody Score and Inmate Security Level 1 Low Security / (Minimum + Low) 0.8 0.6 0.4 0.2 0 0 1 2 3 4 5 6 7 8 9 10 Security Custody Score Notes: Vertical axis measures the share of inmates in low security among those in either minimum or low. That is, if Ms is the number of inmates with score s who are housed in minimum security and Ls is the number who are housed in low security, each datapoint shows Ls Ls + Ms for some score s See Appendix Table 3 for underlying data. 30 Figure 3 Security Custody Score and Rearrest Rates 0.40 Share rearrested within one year 0.35 0.30 0.25 Actual Predicted 0.20 0.15 0.10 0.05 0.00 0 1 2 3 4 5 6 7 8 9 10 Security Custody Score Notes: Vertical axis measures the share rearrested within one year of release. Predicted values are predicted probabilities of rearrest based on a probit model with independent variables: age and dummies for high school graduation status, prior arrest, married, white, male, and employed as of arrest date. 31 5 Appendix: Constructing the Security Custody Score Here, we detail the process by which a prisoner is assigned a security custody score by the bureau of prisons. Upon entry to the federal prison system, an inmate is processed using an Inmate Load and Security Designation Form (see Figure 1). Seven separate items are evaluated by a regional designator for each inmate. Each item is governed by a procedure found in the Bureau of Prisons Security Designation and Custody Classification Manual (Federal Bureau of Prisons, 1982). Discussing each item in the order in which it is addressed on the Designation Form: 5.1 Type of Detainer This category refers to the severity of charges for which the inmate has not yet been tried and sentenced. A pending charge under a state statute would fall under this category, for example. The severity of the worst such charge is ranked from 0 to 7 according to the severity of offense scale (discussed below), and this number becomes the inmate’s type of detainer score, with the exception that 0 means no pending charges, and a score of 1 indicates a pending charge with a severity score of either 0 or 1. 5.2 Severity of Current Offense All offenses are classified according to the Bureau of Prisons Severity of Offense Scale, which exhaustively partitions the penal code into 5 categories: 0 (lowest), 1 (low/moderate), 3 (moderate), 5 (high), and 7 (greatest). The severity of current offense score for an inmate is the severity of the most severe documented behavior associated with the crime for which the individual is currently serving a period of incarceration. For example, if an individual was involved in an armed robbery of a bank (which scores a 7), but plead down at trial to simple robbery (which scores a 5), he would score a 7. 5.3 Expected Length of Incarceration To determine this value the regional designator first looks up the reference (standard) sentence length in months for the inmate, based only on the offense for which the inmate is serving time. These are found in the Expected Length of Incarceration Scale in the Sentencing Handbook. The minimum of this number and the months to which the inmate was actually sentenced is compared to a set of cutoffs, with 0-12 months receiving 0 points, 13-59 receiving 1, 60-83 receiving 3, and 84 or more months receiving 5 points. 32 5.4 Type of Prior Commitments If an inmate has never been incarcerated before he receives a 0. Otherwise, the most severe offense he has been incarcerated for (as evaluated by the severity of current offense scale) is used. An inmate receives 1 point if his most serious prior offense is classified as either low or low-moderate. Any more serious offence conviction leads to a score of 3. 5.5 History of Escape Attempts This measure classifies the escape history of the individual. The history includes a individual’s entire background of escapes or attempts to escape from confinement, excluding the current offense. This includes documented flight to escape prosecution, and if multiple escape attempts were made the most severe is used. The severity of the escape attempt is classified as either minor or serious. A minor attempt must have been from an open institution (work camp, work release, furlough, flight to avoid prosecution) and must not have involved a threat of violence. All other attempts are considered serious. As the security designation form details, this severity and the time elapsed since the attempt, combine to form this score component. 5.6 History of Violence This classifies the violent acts history of the individual. This history comprises a individual’s entire background of violent acts, excluding his current offense. Violent acts enter the history even if noted by a prison discipline committee but never prosecuted. If an inmate has multiple such acts, the most severe is used. The severity of each act is classified as either minor or serious. A minor act is a simple assault, fight, or domestic squabble. Aggravated assault or worse, arson, or any act involving a weapon, or explosives is considered serious. As the security designation form details, this severity and the time elapsed since the act combine to form this score component. 5.7 Pre-Commitment Status An inmate scores 0 if prior to incarceration he was not out on his own recognizance and/or did not voluntarily surrender. He scores -3 if he was released on his own recognizance during his trial without posting bail to ensure appearance, but was incarcerated post-trial. An inmate scores -6 if he meets the previous criteria and surrendered voluntarily to confinement, i.e. was not escorted by a law official to the place of his confinement. 33 Appendix Table 1 Computing the Security Custody Score Inmate characteristic Score Range From To 0 (None) 7 (Greatest) Type of detainer (severity of outstanding charges) Severity of current offense 0 (Lowest) 7 (Greatest) 0 (0-12 Months) 5 (84+ Months) Type of prior commitments 0 (None) 3 (Serious) History of escapes or attempts 0 (None) 7 (Recent Escape) History of violence 0 (None) 7 (Recent Serious) -6 (Voluntary Surrender) 0 (None) 0 36 Expected length of incarceration Precommitment status (bail, bond, etc. set in trial) TOTAL Appendix Table 2 Determining the Appropriate Security Level Score Range Assigned Security Description Level Example 0-6 1 Minimum Danbury Camp 7-9 2 Low La Tuna 10-13 3 Low/Medium Otisville 14-22 4 Medium Petersburg 23-29 5 High Leavenworth 30-36 6 High Marion Source: Federal Bureau of Prisons (1985). 34 Appendix Table 3 Detailed Data Summary Score Number of inmates Percent of inmates in security level: High Percent rearrested within (years): One Two Three Assigned security level based on score: Minimum 0 411 78.35 6.33 2.43 4.87 8.03 4.62 9.98 17.27 1 46 63.04 17.39 6.52 8.70 4.35 17.39 28.26 41.30 2 45 77.78 17.78 0.00 4.44 0.00 26.67 40.00 51.11 3 56 64.29 25.00 1.79 5.36 3.57 19.64 30.36 33.93 4 79 58.23 21.52 10.13 5.06 5.06 24.05 34.18 44.30 5 47 57.45 27.66 0.00 10.64 4.26 17.02 44.68 57.45 6 44 47.73 36.36 6.82 4.55 4.55 22.73 40.91 52.27 Assigned security level based on score: Low 7 31 3.23 54.84 25.81 9.68 6.45 32.26 54.84 61.29 8 20 10.00 65.00 25.00 0.00 0.00 35.00 55.00 65.00 9 33 9.09 18.18 6.06 3.03 27.27 36.36 48.48 Assigned security level based on score: Low/Medium 10 26 3.85 26.92 53.85 15.38 0.00 34.62 61.54 69.23 11 17 11.76 5.88 70.59 5.88 5.88 23.53 23.53 52.94 12 31 3.23 3.23 61.29 29.03 3.23 29.03 45.16 58.06 13 11 0.00 18.18 18.18 54.55 9.09 36.36 45.45 72.73 Min. Low 63.64 Low/Med Medium Assigned security level based on score: Medium 14+ 51 0.00 0.00 11.76 60.78 27.45 29.41 60.78 72.55 ALL 948 55.49 17.30 10.23 10.13 6.86 36.81 16.24 27.32 Note: Inmate percentages may not add to 100 due to rounding error. 35