Law & Policy, Understanding the Place of Punishment, Disadvantage, Politics, and the Geography of Imprisonment in 21st Century America, 2021
Download original document:
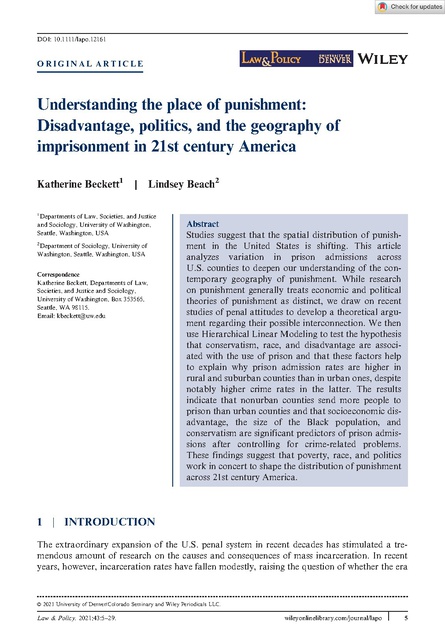
Document text
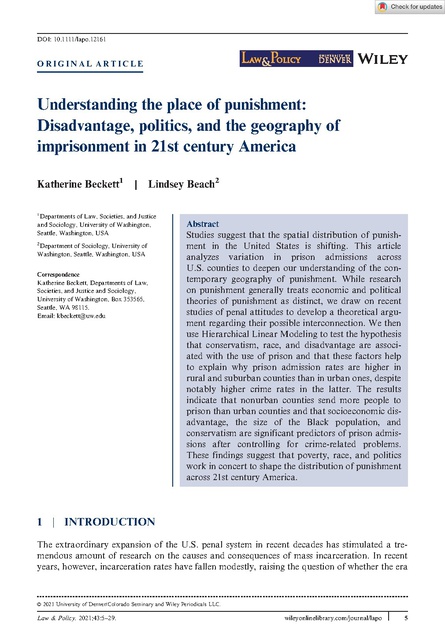
Document text
This text is machine-read, and may contain errors. Check the original document to verify accuracy.
1') Check for updates DOI: 10.1111/lapo.12161 WILEY ORIGINAL ARTICLE Understanding the place of punishment: Disadvantage, politics, and the geography of imprisonment in 21st century America Katherine Beckett1 | Lindsey Beach2 1 Departments of Law, Societies, and Justice and Sociology, University of Washington, Seattle, Washington, USA 2 Department of Sociology, University of Washington, Seattle, Washington, USA Correspondence Katherine Beckett, Departments of Law, Societies, and Justice and Sociology, University of Washington, Box 353565, Seattle, WA 98115. Email: kbeckett@uw.edu Abstract Studies suggest that the spatial distribution of punishment in the United States is shifting. This article analyzes variation in prison admissions across U.S. counties to deepen our understanding of the contemporary geography of punishment. While research on punishment generally treats economic and political theories of punishment as distinct, we draw on recent studies of penal attitudes to develop a theoretical argument regarding their possible interconnection. We then use Hierarchical Linear Modeling to test the hypothesis that conservatism, race, and disadvantage are associated with the use of prison and that these factors help to explain why prison admission rates are higher in rural and suburban counties than in urban ones, despite notably higher crime rates in the latter. The results indicate that nonurban counties send more people to prison than urban counties and that socioeconomic disadvantage, the size of the Black population, and conservatism are significant predictors of prison admissions after controlling for crime-related problems. These findings suggest that poverty, race, and politics work in concert to shape the distribution of punishment across 21st century America. 1 | INTRODUCTION The extraordinary expansion of the U.S. penal system in recent decades has stimulated a tremendous amount of research on the causes and consequences of mass incarceration. In recent years, however, incarceration rates have fallen modestly, raising the question of whether the era © 2021 University of Denver/Colorado Seminary and Wiley Periodicals LLC. Law & Policy. 2021;43:5–29. wileyonlinelibrary.com/journal/lapo 5 6 UNDERSTANDING THE PLACE OF PUNISHMENT of mass incarceration is coming to a close. At the same time, the geography of punishment has been undergoing notable change, as urban areas increasingly embrace criminal justice reform while many nonurban jurisdictions maintain or intensify their use of jail and prison (Beckett & Beach, 2020; Kang-Brown et al., 2018; Keller & Pearce, 2016; Simes, 2018; Subramanian et al., 2015). Evidence of changes in the spatial distribution of punishment has emerged alongside growing recognition of the increasingly divergent economic fates and political ideologies of urban and nonurban communities (e.g., Edsall, 2017; Grusky et al., 2011). Although urban areas largely recovered from the economic downturn of the mid-2000s, this is not true of many rural communities, which are also grappling with the effects of the opioid crisis (Mather & Jarosz, 2016). Poverty and attendant problems have also been rising in some suburban locales (Kneebone, 2017; Kneebone & Berube, 2013). At the same time, the chasm between the political orientations of rural and urban areas has grown ever larger (Edsall, 2017; Kron, 2012). In short, shifts in the geography of punishment are occurring at the same time that the economic fates and political orientations of urban and nonurban areas increasingly diverge. Geographic variation in the use of prisons in 21st century America thus affords an opportunity to assess—and advance—alternative theoretical perspectives on punishment. One well-known school of thought emphasizes the role played by concentrated disadvantage as a determinant of “incarceration hot spots” at the local level (Sampson, 2012; Sampson & Loeffler, 2010; see also Simes, 2018). These studies of spatial variation in incarceration rates find a high degree of correlation between crime rates and disadvantage, but also between disadvantage and incarceration. In Chicago, for example, concentrated disadvantage is a significant predictor of incarceration rates independent of its association with crime (Sampson & Loeffler, 2010, p. 28). While most of this literature focuses on urban neighborhoods, Simes (2018) also finds that concentrated disadvantage is significantly and positively correlated with incarceration rates at the census tract level across the state of Massachusetts. The independent association of disadvantage and punishment has thus been found to exist in numerous locales, but it is not well understood. Another line of research emphasizes the role of political ideology in shaping penal outcomes, analyzing variation in incarceration-related outcomes at the state and county levels to assess its import. These studies indicate that the strength of the Republican Party and other measures of the conservativism of local constituents are strongly predictive of intensified punishment and spending on social control. Consistent with the predictions of the racial threat perspective, many of these studies also find that the size of the Black population is significantly and positively correlated with incarceration rates after controlling for crimerelated problems (Beckett & Western, 2001; Helms and Jacobs, 2002; Jacobs & Carmichael, 2001; Jacobs & Helms, 1999; Jacobs & Jackson, 2010; Weidner & Frase, 2003; Western, 2006). In what follows, we describe the changing geography of social disadvantage, political ideology, race and punishment in 21st-century America. We then describe various theories of punishment in greater detail and develop a theoretical account of the independent impact of disadvantage on incarceration. Specifically, drawing on recent studies of penal attitudes, we develop and assess the hypothesis that disadvantage, race, and conservativism work together to explain geographic variation in the use of prisons. To accomplish these goals, we employ a multilevel modeling strategy to analyze an original data set that includes information about crime, arrests, socioeconomic disadvantage, demographic characteristics, political ideology, substance abuse, and prison admissions at the county level. Hierarchical Linear Modeling (HLM) enables analysis of these county-level characteristics while correcting for the clustering of counties within states. The conclusion summarizes the findings and considers their theoretical and policy implications. LAW & POLICY Ni-WILEYJ_ 7 2 | SHIFTING FORTUNES? RECENT DEVELOPMENTS IN RURAL, SUBURBAN, AND URBAN AMERICA Recent research shows that jails have expanded far more rapidly in small and medium-sized counties than in large urban counties. In fact, a plurality (44%) of jail inmates are incarcerated in small counties (Kang-Brown et al., 2018; Subramanian et al., 2015). Similarly, it appears that in recent years prison admissions have fallen in large counties but risen in small counties (Kang-Brown et al., 2018; Keller & Pearce, 2016). Although these descriptive studies provide evidence of increased geographic divergence in jail and prison admissions, little is known about why this spatial shift is occurring. Alternative theoretical perspectives on punishment suggest several potential explanations. 2.1 | Concentrated disadvantage and incarceration One theoretical perspective emphasizes the link between socioeconomic disadvantage, crime, and incarceration. From this perspective, the spatial concentration of disadvantage (measured in terms of poverty, unemployment, family disruption, and spatial segregation) fuels violence and involvement in illicit markets, which increases incarceration rates, which in turn exacerbates both crime and disadvantage (see especially Sampson, 2012; Sampson & Loeffler, 2010). But these studies also show that disadvantage has its own independent effects on incarceration after controlling for crime. In Chicago, for example, some neighborhoods with high levels of disadvantage experience incarceration rates that are more than three times higher than other communities with similar crime rates but lower levels of disadvantage (Sampson & Loeffler, 2010; Sampson, 2012). From this perspective, then, incarceration is both a reflection and cause of concentrated disadvantage (Sampson, 2012, 2014; Sampson & Loeffler, 2010; see also Simes, 2018; Vankatesh and Levitt, 2000). As noted previously, the empirical work that supports this theoretical framework focuses mainly on neighborhood-level variation in incarceration across large cities, especially Chicago. Yet the theoretical emphasis on the role of concentrated disadvantage has broader applicability, and suggests the possibility that increased penal severity in rural and suburban areas in the 21st century may reflect changes in the spatial distribution of social marginality. Indeed, there is evidence that the geography of poverty and marginality have changed markedly in recent years, and especially in the aftermath of the Great Recession of 2007–2009 (hereafter “the recession”). Specifically, disadvantage has deepened in rural America both in absolute terms and relative to urban areas (Lichter et al., 2012). For example, the number of nonmetropolitan communities with poverty rates of 30% or higher increased by 50% during and after the recession (Lichter et al., 2012). The proportion of rural counties that experience high rates of both poverty and inequality is now more than twice as high as the share of large metropolitan counties characterized by these twin markers of disadvantage (44% vs. 21%) (Mather & Jarosz, 2016). Whereas employment rates in metropolitan areas have recently been significantly higher than they were prior to the recession, employment in nonmetropolitan areas remains well below prerecession rates (Edsall, 2017). Similarly, the rural–urban gap in both poverty and child poverty rates began to grow following the recession. By 2012, the rural child poverty rate had climbed to over 26%, significantly higher than the urban child poverty rate of 21% (Farrigan, 2014). And while rates of deep poverty (defined as having cash income that is less than half of the poverty threshold) have historically been comparable in rural and urban areas—hovering around 5% for much of the 1980s and 1990s—in the aftermath of the Great Recession, more than 12% of all rural children lived in deep poverty compared to just under 9% of urban children (Farrigan, 2014). 8 UNDERSTANDING THE PLACE OF PUNISHMENT Sociologists have identified a number of other socioeconomic trends that, like poverty, increasingly characterize the “new rural America” (Burton et al., 2013). For example, particularly in the aftermath of the Great Recession, many rural communities experienced population loss, especially among young adults, as the geographically mobile increasingly relocated to metropolitan areas in search of employment and other opportunities (Burton et al., 2013). At the same time, rising joblessness among men combined with increasing levels of female employment have fueled a notable rise in single parent households in these communities (Burton et al., 2013). The health and well-being of many rural residents has declined in this context (Burton et al., 2013), and the injury-related mortality rate is now notably higher in rural areas (Myers et al., 2013). Recent evidence of a sharp rise in “deaths of despair” among middle-aged white men and women (Case & Deaton, 2015) may reflect, at least in part, this recent transformation of rural life.1 In short, recent studies suggest that various manifestations of social disadvantage have intensified in rural areas. Many suburban areas are also undergoing significant transformation, with the number of suburbanites living in poverty growing by 57% from 2000 to 2015 (Kneebone, 2017). By 2010, 55% of the nation’s poor were concentrated in the suburbs (Kneebone & Berube, 2013). This “suburbanization of poverty” was driven in part by the relocation of poor urban residents fleeing gentrification, as well as by the implementation of HOPE IV, a federal government program that integrates poor housing voucher recipients into mixed income communities (Lacy, 2016). The geographic dispersal of employment opportunities, especially low-paid jobs, outside of central cities also fueled suburban growth and poverty (Raphael & Stoll, 2010). Notably, suburban poverty has increased not only in older, distressed inner-ring suburbs but also in more far-flung suburban and exurban communities (Kneebone, 2017). The intensification of poverty and disadvantage in nonurban areas has been accompanied by shifts in the spatial distribution of crime. Historically, crime has been disproportionately concentrated in urban counties (Wells & Weisheit, 2004). While this pattern persists, the gap between rural, suburban, and urban areas has narrowed in recent years. For example, national victimization survey data indicate that the violent crime rate fell by 40% in urban counties but by just 25% and 18% in suburban and rural areas (respectively) from 2005 to 2014 (Truman & Langton, 2015). At the same time, substance abuse, and the misuse of opioids in particular, has increased especially rapidly in nonurban areas (Keyes et al., 2014; Love et al., 2016). This latter shift may reflect, in part, the recent dispersal of drug trafficking organizations to rural, suburban, and fringe metropolitan communities, as well as the intensification of human suffering in these communities (Quinones, 2015; Reding, 2009). In short, the spatial distribution of disadvantage has shifted in recent years, as poverty and related problems have become more pronounced in nonurban areas. Theoretical perspectives that highlight the relationship between disadvantage, crime, and incarceration suggest that more disadvantaged counties will also send significantly more people to prison after crimerelated factors are taken into account; this pattern may help explain the geography of punishment in 21st century America. While the empirical associations between crime, disadvantage, and incarceration have been amply documented, the independent association of concentrated disadvantage and incarceration remains poorly understood. Below, we discuss the relationship of political ideology and race to punishment, and consider how these dynamics might also help to explain why disadvantage is associated with intensified punishment even after crime rates are taken into account. 2.2 | Social control, race, and politics Some analysts emphasize the politics of social control to explain the distribution of punishment over time and across space. Broadly speaking, social control theorists posit that punishment, LAW & POLICY Ni-WILEYJ_ 9 and U.S. mass incarceration in particular, reflects racial and/or political dynamics that are somewhat independent of crime trends (Alexander, 2010; Beckett, 1997; Beckett & Francis, 2021; Gottschalk, 2015; Kohler-Hausman, 2017; Weaver, 2007; Western, 2006). One version of this approach, the racial threat perspective, holds that large or growing Latinx or Black populations trigger (White) fear that results in enhanced punishment. Many studies find evidence of this empirical association (Beckett & Western, 2001; Blalock, 1967; Greenberg & West, 2001; Jacobs & Carmichael, 2001; King, 2007; Liska et al., 1982; Weidner & Frase, 2003), suggesting that the recent uptick in incarceration in rural and suburban areas may be related to recent demographic shifts. Although some of America’s most impoverished communities of color have long lived in geographically isolated rural areas such as the Mississippi Delta and on Native lands (Burton et al., 2013), rural areas have historically been less racially diverse than their urban counterparts (Housing Assistance Council, 2012). In recent years, however, new immigrants have increasingly settled in unconventional destinations, including some small towns across rural America, notably transforming the demographic and social fabric of these communities (Donato et al., 2008; Lichter et al., 2012; Massey & Capoferro, 2008). Indeed, the dispersion of mainly Latinx immigrant families into new rural destinations, especially in the Midwest and Southeast, has increasingly offset the exodus of whites from at least some of those communities (Donato et al., 2008). Like rural areas, suburbs are increasingly home to immigrants and their children. Moreover, recent immigrants have tended to settle in suburbs and small towns immediately upon arrival, bypassing the urban enclave as the first destination for newcomers (Donato et al., 2008; Massey & Capoferro, 2008). During the 2000s, 80% of the population growth that occurred in suburbs was attributed to immigrants (Suro, Wilson, and Singer, 2011). By 2010, the majority (51%) of the immigrant population was living in the suburbs of the nation’s largest metropolitan areas (Singer, 2011). As in rural communities, these and other demographic shifts have had a pronounced impact on community relations and life in suburban communities (Lacy, 2016). The racial threat perspective highlights the possibility that these shifts—specifically, the growth of the immigrant population in nonurban settings—may be fueling support for punitive practices that result in high prison admission rates. This hypothesis is consistent with sociolegal studies indicating that legal sanctions are often mobilized by communities seeking to reinforce traditional arrangements in the context of the arrival of “outsiders”. It is also consistent with evidence that support for punitive policies increases in the context of heightened immigration and concerns about declining social cohesion (Tyler & Boekmann, 1997). Social control perspectives also emphasize the impact of political ideology on penal discourse and outcomes. From this perspective, Punishment should be seen not in the narrow terms of the “crime problem” but instead as one of the mechanisms for managing the underclass. . . . In this broader view, criminal penal measures are shaped not just by patterns of criminality, but primarily by governmental perceptions of the poor as social problems and the preferred strategies for their treatment. (Garland, 1990, p. 134) Consistent with this view, social control theorists focusing on the contemporary United States specifically highlight the utility of the crime issue in conservative governance strategies developed in response to the civil rights movement and associated changes in the electoral landscape (Beckett, 1997; Beckett & Francis, 2021; Davey, 1998; Jacobs & Jackson, 2010; Weaver, 2007). For conservatives seeking to mobilize and attract voters alienated by the Democratic Party’s embrace of the civil rights cause after 1964, the crime issue was an especially useful means of building political coalitions that supported harsh criminal punishment, welfare retrenchment, and other “tough” approaches to poverty-related problems (Beckett, 1997; 10 UNDERSTANDING THE PLACE OF PUNISHMENT Beckett & Francis, 2021; Kohler-Hausman, 2017; Soss et al., 2011; Weaver, 2007). From this perspective, then, the politicization of the crime issue and the embrace of “tough” anticrime policies in the aftermath of the 1960s were not only a response to the civil rights movement and the electoral dynamics it generated, but also part of a conservative effort to enhance the state’s social control capacity while weakening its commitment to social welfare.2 Consistent with this perspective, studies analyzing state-level variation in punishment rates have generally found that the strength of the Republican party and/or conservative values are significant predictors of incarceration rates and spending on social control (Beckett & Western, 2001; Jacobs & Carmichael, 2001; Jacobs & Helms, 1999; Jacobs & Jackson, 2010; Western, 2006).3 Jacobs and Carmichael (2001) identify two mechanisms that may explain the relationship between Republican Party strength and incarceration. First, Republicans in particular have drawn for several decades on a racialized rhetoric that tends to magnify public demands for severe penalties (see also Beckett, 1997; Davey, 1998; Weaver, 2007). It is therefore likely that this rhetoric is more widespread in jurisdictions characterized by a strong Republican presence. In addition, after gaining office, Republican officials often impose harsher sentences and enhance prison capacity. There is also evidence that conservative citizen ideology (independent of the strength of the Republican party) predicts higher incarceration rates, presumably because conservatives tend to emphasize the individual rather than social determinants of behavior and are more likely to believe that more severe penalties will deter would-be law violators (Jacobs & Carmichael, 2001). This body of research demonstrates the centrality of both political ideology and race (especially Blackness) to the distribution of punishment and suggests that the shift in the geography of punishment in the United States may be bound up with larger changes in the political landscape. Whereas political divisions historically fell along state and regional lines, “The new political divide is a stark division between cities and what remains of the countryside” (Kron, 2012). This split has been growing for some time: an increasing number of America’s major cities have voted Democratic each year over the past several decades. For example, only four large cities (Phoenix, Oklahoma City, Fort Worth, and Salt Lake City) voted Republican in the 2012 Presidential election; all others—including those located in famously red states such as Texas—voted Democratic. This pattern was even more pronounced in the 2016 Presidential election (Badger et al., 2016). The simultaneous intensification of the rural/urban political and penal divides suggests that the increased use of prison and jails in nonurban areas may reflect broader shifts in the political landscape. That is, the increased use of jails and prisons in nonurban areas may reflect the intensification of conservatism in those areas, which in turn has implications for the ideological orientation of justice officials. In fact, the vast majority of prosecutors and judges are elected in the United States. These elected officials possess a good deal of discretion, the exercise of which has a pronounced impact on penal outcomes (Davis, 2008; Lynch, 2016; Pfaff, 2017; Stuntz, 2011). Prosecutorial discretion, for example, is enormously consequential: prosecutors significantly impact criminal justice outcomes by altering their charging practices or modifying their standards for plea bargains (Davis, 2008; Lynch, 2016; Pfaff, 2017; Stuntz, 2011). The hypothesis that diverging penal trends in urban and nonurban areas reflect increasingly disparate political orientations is thus consistent with theoretical perspectives that underscore the important influence of local norms on penal practices and outcomes (Campbell & Schoenfeld, 2013; Lynch, 2011, 2016). In sum, social control theorists see punishment as a key component of state strategies for managing the poor and marginalized, and therefore emphasize the role of politics, racial dynamics, and political ideology as determinants of penal outcomes. This body of literature has been largely distinct from research emphasizing the impact of concentrated disadvantage on incarceration-related outcomes. However, insofar as punishment is a component of state strategies for governing the poor, this separation may be unwarranted. Below, we draw on research LAW & POLICY Ni-WILEYJ_ 11 that illuminates the impact of politics on penal outcomes—as well as recent studies of penal attitudes—to develop a theoretical account of the independent association of concentrated disadvantage and incarceration. 2.3 | Disadvantage, race, politics, and punishment: Understanding the connections As noted previously, many studies have found that the size of Black and/or minority populations is positively associated with incarceration rates (Beckett & Western, 2001; Blalock, 1967; Greenberg & West, 2001; Jacobs & Carmichael, 2001; King, 2007; Liska et al., 1982; Weidner & Frase, 2003). While quantitative studies are generally unable to identify the mechanisms that explain this association, the racial threat perspective holds that comparatively large minority populations pose a threat to, and engender fear and bias on the part of, White residents; these sentiments are manifested in the greater use of prisons (Blalock, 1967). However, some studies also suggest that this effect diminishes when the Black population is comparatively large, possibly reflecting the greater political power of the Black community in those jurisdictions (Keen & Jacobs, 2009). Thus, the relationship between the size of the Black population and prison admissions may be nonlinear. Research showing that the size of the Black population influences rates of incarceration for non-White residents, but not White residents, provides support for the racial threat hypothesis (Bridges et al., 1987). Similarly, we suspect that comparatively high levels of concentrated disadvantage trigger concern and fear on the part of more affluent residents, to which elected criminal justice officials feel compelled to respond. This hypothesis emphasizes that the greater presence of stigmatized groups (including Black people and people living in poverty) triggers bias, and is thus distinct from Marxian arguments regarding elites’ use of the criminal justice system to manage the unemployed and others who pose a threat to the social order. Many studies have examined this Marxian hypothesis and found weak or no support for it (see Jacobs & Helms, 1999; Western, 2006 for a discussion). There are additional theoretical grounds for anticipating that conservatism, race, and disadvantage help to explain why prison admission rates are comparatively high in nonurban counties. Recent research shows that animus toward the poor (measured mainly in terms of opposition to welfare) is an important predictor of punitive attitudes regarding criminal punishment (Brown & Socia, 2017). This research also indicates that anti-Black affect, animus toward the poor, and support for harsh criminal penalties tend to cluster. Thus, support for conservative penal policies and racial animus are associated with opposition to welfare spending and other measures aimed at leveling the playing field. Similarly, Helms and Jacobs (2002) found that African-American defendants receive longer sentences than similarly situated others in conservative counties, suggesting that bias is more pronounced (or at least more likely to be expressed in criminal sentences) in more conservative jurisdictions. For these reasons, we hypothesize that conservatism, the size of the Black population, and disadvantage help explain higher prison admission rates in nonurban counties after taking crime and other relevant factors into account. 3 | DATA AND METHODS Our analysis is designed to explore the impact of various county characteristics on prison admissions in order to assess the viability of alternative theories of punishment. More specifically, we seek to determine whether conservatism, race, and disadvantage help to explain the spatial distribution of prison admissions in counties across the United States. To accomplish 12 UNDERSTANDING THE PLACE OF PUNISHMENT these goals, we constructed and analyzed an original, county-level data set, which we describe below. 3.1 | Data Our multilevel regression analysis identifies the determinants of admissions to state prisons, where the majority of U.S. inmates reside.4 The starting point for our analyses is the “iron law of prison populations” (Clear & Austin, 2009), which holds that imprisonment rates are a function of two factors: prison admissions and the amount of time people spend in prison. The latter is largely determined by the sentence imposed as well as a variety of postconviction practices and policies, especially state-level policies regarding parole release and the ability of prisoners to earn reductions to their sentences. Given our interest in local, county-level differences in the use of prisons, we focus on prison admissions over which county officials have influence, that is, prison admissions stemming from the commission of crimes or violations of the terms of probation. Admissions stemming from technical parole violations are determined by state correctional authorities, and are therefore not included in our analyses (see also Blumstein & Beck, 1999; Neal and Rick, 2014; Raphael & Stoll, 2013; Travis et al., 2014). We include prison admissions involving persons sentenced to prison for twelve months or more.5 Data pertaining to prison admissions are taken from the National Corrections Reporting Program (NCRP). The NCRP provides individual-level, administrative data collected by the Bureau of Justice Statistics that include demographic information, offense type, length of sentence imposed, county of sentencing, type of admission, and type of release. The collection began in 1983 and is available through the Inter-University Consortium for Political and Social Research at the University of Michigan. NCRP data are available for counties in twenty-eight states from 2013, but for notably fewer states in 2014. For this reason, our dependent variable is the prison admission rate observed in 2013. Data regarding independent variables are drawn from a variety of sources. We use the detailed categorization scheme provided by the Centers for Disease Control and Prevention’s National Center for Health Statistics (NCHS) to identify urban, suburban, and rural counties (see Ingram & Franco, 2014).6 The NCHS’s categorization scheme includes total population and density measures as well as information about counties’ metropolitan/nonmetropolitan status, the size of the surrounding metropolitan or micropolitan statistical area, and demographic and socioeconomic information. Although large central metropolitan (i.e., urban) counties and large fringe metropolitan (i.e., suburban) counties may have similar population sizes, NCHS analysis suggests that these counties differ significantly in terms of other social characteristics (Ingram & Franco, 2014, p. 4). We therefore include large central metropolitan counties in the urban category, while large fringe, medium, and small metropolitan counties are classified as suburban. Micropolitan and noncore (i.e., rural) counties have smaller population centers and are not economically and socially connected to larger metropolitan areas. To be sure that the three-category classification system we used was not unduly influencing the results, we also ran the models using a four-category measurement system in which large fringe and medium metropolitan counties were classified as large suburban counties while small metropolitan counties were classified as small suburban counties. The findings of the stepwise models that included all four geographic categories were similar in size, direction, and significance as those reported here. Crime and arrest data are taken from the FBI’s Uniform Crime Reports (UCR)7 for 2010–2012. These data are aggregated by the National Archive of Criminal Justice Data to the county level. UCR data identify twenty-eight different offenses, eight of which are considered comparatively serious. Of these eight “index crimes,” four—murder and nonnegligent manslaughter, rape, aggravated assault, and robbery—are categorized as violent, while another LAW & POLICY Ni-WILEYJ_ 13 four—burglary, larceny-theft, motor vehicle theft, and arson—are classified as property crimes. We focus on these comparatively serious index crimes, all of which have the potential to become felony cases and, therefore, to trigger prison admission. We also include arrests for weapons violations, which can also trigger felony charges, as well as all drug offenses other than marijuana possession (because marijuana possession arrests very rarely result in prison admission).8 The majority of counties now report data to the UCR. For example, in 2012, the law enforcement agencies participating in the UCR program represented more than 270 million U.S. inhabitants—more than 85% of the total population. To ensure the quality and reliability of UCR data, counties with fully imputed data and/or counties in which the difference in sampled populations between offense and arrest data was greater than 10% were dropped from the analysis. We employ a variety of socioeconomic indicators to measure social disadvantage, including the share of the population with income below the poverty threshold, the proportion of the population over twenty-five years of age that has not completed high school, the percentage of households that are female-headed, the proportion of the civilian labor force that is unemployed, and the age-adjusted premature mortality rate. These data are taken from the U.S. Census’ 2012 Small Area Income and Poverty Estimates, the 2012 American Community Survey’s 5-year estimates, and the Centers for Disease Control. These measures are included in a single-concentrated disadvantage index derived using principal component analysis. Analysis confirms the emergence of a single factor that explains 62% of the variance. All index variables load positively on the component, ranging from a factor loading of 0.5 for percentage of the population living in poverty and 0.33 for percentage of the labor force that is unemployed (see Appendix A). High values for the concentrated disadvantage index indicate that a county is experiencing high levels of poverty and related social problems; small or negative values indicate that a small share of the county population is experiencing concentrated disadvantage. Information about county-level demographics including race, ethnicity, total population size, and nativity are taken from the American Community Survey and the U.S. Census. We used the change in the percentage of the population that identified as non-Hispanic White from 2000 to 2012 as indicators of racial threat. The share of the population identifying as Black and the Black share of the population squared are also included in our models, given previous findings regarding the potential impact of these variables. The basic racial threat hypothesis is tested using percent Black and percent Black squared. As Keen and Jacobs explain, “including a squared term captures the possibility that the effect of the percent black population on prison admissions may increase (or decrease) in a nonlinear way as a county’s black population changes. While we could imagine the percent Black increasing the rate of prison admissions in a county, the racial threat hypothesis also suggests that this effect may diminish over time as the black population increases” (Keen & Jacobs, 2009, p. 215). Two center for disease control (CDC) measures of the extent of drug use are also included in the models: the opioid prescription rate in 2012 (per 100 residents) and the average drug-death mortality rate between 2008 and 2012 (per 100,000 residents) (see Monnat, 2018 and Appendix A for more information about these measures). The conservatism of the local political climate is measured by the 2012 presidential election results, and specifically as the share of the county population that voted for the Republican presidential nominee. These data were compiled by American University9 and The Guardian (Rodgers & Cage, 2012); we also supplemented these sources with voting data provided by CNN.10 Missing data for independent variables were limited; where data were missing, they were replaced using multiple imputation methods with predictive mean matching (see Appendix B for more information about data sources and measurement). The sample includes 2333 counties—fifty urban, 835 suburban, and 1448 rural—in thirtyfive states, comprising 74% of all U.S. counties. The states included are regionally diverse and highly varied in terms of population size and density. The states and the number of counties included in the sample are listed in Appendix C. UNDERSTANDING THE PLACE OF PUNISHMENT 14 3.2 | Analytic strategy and methods We use HLM to examine the association of county-level covariates with the 2013 logged prison admission rate. Because we are interested in county-level variation in the use of prisons, we control for the effects of state differences but do not seek to identify specific state-level drivers of prison admissions.11 A two-level hierarchical linear model “nests” cases (counties) within groups (states) to statistically account for clustering in the prison admission rate, providing more useful comparisons between counties in differing states. We address this state clustering of prison admission rates using random intercepts for each state (β0j). While inclusion of dummy variables in ordinary least squares (OLS) regression would also enable us to control for the effects of state-level factors, HLM also enables the estimation of county-level effects related to variation in prison admissions by accounting for the error structure at each level. This helps to correct downward biased estimates of SEs due to nesting (Raudenbush and Bryk, 2002). However, to ensure that this methodological choice did not unduly influence the results, Appendix D shows a second set of results from OLS regressions with state-level dummies. These results are very similar to those shown in the main text. Consistent with our theoretical framework, we sequentially introduce variables to the base random-intercept model. The first set of control variables includes jurisdiction type (β1County Typeij), and the second set includes crime and policing variables (β2Offense Rateij, β3log[Drug Arrest Rate]ij and β4Arrest:Offenseij). We then introduce the concentrated disadvantage index and measures of drug abuse in the third model (β5Con. Dis. Indexij, β6log[Drug-Related Death Rate]ij, and β7Opioid Prescribing Rateij), followed by county-level measures for the racial threat hypothesis—(β8% Blackij, β9% Black)2ij, and (β10Change Whiteij)— and the political ideology variable (β11% Republican Voteij). For county i in state j, we fit the following regression to the log prison admission rate: logðPrison Admission RateÞij = β0j + β1 County Typeij + β2 Offense Rateij + β3 logðDrug Arrest RateÞij + β4 Arrest : Offenseij + β5 Con:Dis:Indexij + β6 logðDrug-Related Death RateÞij + β7 Opioid Prescribing Rateij + β8 %Blackij + β9 %BlackÞ2ij + β10 Change Whiteij + β11 %Republican Voteij and β0j = γ 00 + μ0j : Here, γ 00 is the average log prison admission rate for the sample population, μ0j is the statespecific effect on the log prison admission rate, and εij is the Level 1 (state) residuals. Restricted maximum likelihood methods (REML) were used in all models. Highly skewed variables were transformed where appropriate, and assessments of multicollinearity (measured through variable inflation factor tests) informed model selection. Leverage tests were conducted to ensure that outlying counties did not significantly impact our overall estimates. Z-score transformations were performed (mean = 0, SD = 1) to compare effects across all explanatory variables and to standardize variables with divergent scales. Due to variable scaling and the logged transformation of the dependent variable, regression coefficients were interpreted as the percent change in the logged prison admission rate for a 1 SD increase in a predictor, holding all other variables constant. Poisson and negative binomial model specifications for a county-count of prison admissions were also explored for basic and final model specifications. Both specifications performed significantly less well than the final linear specification. We also explored spatial autocorrelation, as Simes (2018) found significant spatial autocorrelation between Massachusetts census tracts, for which she corrected by including a spatial lag in her model. To test for possible spatial LAW & POLICY Ni-WILEYJ_ 15 autocorrelation within states, we compared two basic models: M1, with no correction for within-class correlation, and M2, with the correlation matrix specified with a compound symmetry structure (corresponding to a constant correlation). The log likelihood for both models was calculated, subtracted (M2 – M1), and multiplied by 2 to calculate the G2 statistic, which was not statistically significant when analyzed using a χ 2 distribution. For this reason, spatial lags are not included in our models. 4 | FINDINGS The descriptive statistics associated with the samples included in the analyses are shown in Table 1. These data identify a number of patterns that may contribute to geographic variation in the use of prisons across county types. Although urban counties in our sample have, on average, comparatively high crime and drug arrest rates, rural counties are more conservative, have TABLE 1 Descriptive statistics (mean, SD, and range) by county type Variable Period Urban Suburban Rural Prison admission rate 2013 133.31 (67.0) 28.5–324.8 149.61 (92.4) 10.7–726.7 167.93 (120.7) 6.6–1149.4 Prison admission rate (logged) 2013 4.755 (0.56) 3.35–5.78 4.821 (0.64) 2.37–6.59 4.88 (0.75) 1.89–7.05 Felony offense rate 2010–2012 4412 (1528) 2350–9868 2738 (1233) 16.46–7214 2127 (1329) 0–15,927 Felony drug arrest rate (logged) 2010–2012 5.70 (0.58) 4.49–7.53 5.13 (0.72) 0–8.42 4.86 (1.11) 0–8.28 Arrest to offense ratio 2010–2012 0.18 (0.09) 0.06–0.42 0.19 (0.08) 0–0.9 0.21 (0.13) 0–1.95 Con. disadvantage index 2012 0.65 (1.47) −2.51 to 5.03 −0.27 (1.49) −3.55 to 5.15 0.16 (1.85) −3.43 to 6.99 Drug-related death rate (logged) 2008–2012 2.63 (0.42) 1.75–3.42 2.59 (0.43) 1.11–3.96 2.72 (0.47) 1.40–4.61 Opioid prescribing rate 2012 74.04 (24.01) 28.8–141.2 92.58 (41.5) 0.5–335.1 98.69 (50.55) 4.9–387.2 % Black 2012 22.05 (13.8) 1.6–63.7 9.31 (12.14) 0–74 6.89 (13.51) 0–84.5 Change in % non-Hispanic White 2000 & 2012 −6.03 (3.32) −13.1 to 1.95 −3.86 (3.5) −31.39 to 6.33 −2.71 (3.03) −38.96 to 6.16 % Republican presidential vote 2012 (Romney) 37.02 (14.34) 8.3–65.1 54.72 (14.56) 9.2–90.9 59.94 (15.82) 11.6–95.9 50 835 1448 Sample size 16 UNDERSTANDING THE PLACE OF PUNISHMENT higher arrest-to-crime ratios (a measure of the strength of the police response to crime) and opioid prescribing rates, and have the highest level of disadvantage in the dataset. Although much of the literature highlights the changing demography of rural communities, White populations shrunk more in urban than rural communities in the counties included in our sample. zThe results of the stepwise HLM analysis reveal that rural and suburban counties have significantly higher prison admission rates than urban counties, and these differences remain significant after all variables are included in the model (see Table 2). All three crime and arrest variables are statistically significant in the models. Specifically, the felony offense rate, the drug arrest rate, and the arrest-to-crime ratio have a significant and positive impact on prison admission rates. In addition, concentrated disadvantage is a strong and significant predictor of prison admission rates. After all variables are included in the model, an increase of 1 SD in the concentrated disadvantage index results in a 21% increase in the log prison admission rate across all county types. Relatedly, the opioid prescription rate is a statistically significant predictor of prison admissions. Holding all other variables constant, a 1 SD increase in the opioid prescription rate results in a roughly 7% increase in the average logged prison admission rate in the final models. The size of the Black population is also a significant predictor of prison admissions, although this relationship appears to be nonlinear. After controlling for county-type, crime, and poverty, a 1 SD increase in the percentage of the county population that is Black results in an 8.6% increase in the logged prison admission rate; this finding persists, and its magnitude increases slightly, after our measure of political conservatism is introduced into the model. The nonlinear measure of the Black population is also significant, albeit negative. This latter finding indicates that the effect of the size of the Black population on prison admissions diminishes as the Black population increases in a county. That is, the size of the Black population matters more in smaller, rural counties than in larger urban ones. Change in the non-Hispanic white population has a nonsignificant effect on prison admissions throughout all models. Finally, conservatism has a highly significant impact on prison admissions. Specifically, increasing the share of the Romney vote by 1 SD results in a 10% increase in the logged prison admission rate, all other factors being equal. County-level political ideology thus has an important impact on prison admissions. These results indicate that factors highlighted by theories of concentrated disadvantage and social control are significant predictors of the use of prisons across county types. In particular, disadvantage, race, and political orientation have a highly significant impact on prison admissions across U.S. counties in the post-recession context. 5 | DISCUSSION AND CONCLUSION The results of the HLM analysis identify a number of county-level characteristics that have a significant impact on prison admissions in the post-recession context. Several control variables such as crime rates and drug arrests are, as expected, positively and significantly associated with prison admissions at the county level. After controlling for these factors, concentrated disadvantage, the opioid prescribing rate, the size of the Black population, and political orientation (i.e., support for the Republican presidential candidate in 2012) are also significantly associated with prison admissions. These findings provide support for both theories of concentrated disadvantage (which highlight the independent impact of disadvantage and related problems as a driver of incarceration) and social control perspectives (which foreground the impact of racial dynamics and political ideology on penal outcomes). More generally, these findings are consistent with theoretical perspectives that underscore the importance of local influences on penal practices and outcomes (Campbell & Schoenfeld, 2013). Although state contexts explain a significant portion of the variation in the use of prisons observed across counties, local variation is also pronounced. This is consistent with sociolegal scholarship that highlights the role of 0.172 (0.014) 0.109 (0.014) 0.046 (0.013) — — — — — — — — — — — — — — — — Felony drug arrest rate (logged) Arrest to offense ratio Concentrated disadvantage index Drug-related death rate (logged) Opioid prescribing rate % Black % Black (squared) Change in % non-Hispanic White % Romney vote 0.241 (0.080) — 0.639 −0.039 (0.083) Suburban 0.346 (0.081) — 4.444 (0.108) Felony offense rate 0.970 0.003 (0.083) — County type (Urban) *** Rural 4.750 (0.112) β (SE) β (SE) p Crime County type HLM results: County-level determinants of prison admission rates, 2013 (Intercept) Fixed effects TABLE 2 *** *** *** ** *** *** p — — — — 0.093 (0.001) 0.006 (0.013) 0.151 (0.017) 0.021 (0.012) 0.092 (0.014) 0.107 (0.015) 0.227 (0.079) 0.235 (0.079) — 4.544 (0.101) β (SE) Poverty *** 0.644 *** 0.091 *** *** ** ** *** p — −0.014 (0.012) (0.032) −0.140 0.086 (0.039) 0.081 (0.018) 0.006 (0.013) 0.184 (0.019) 0.018 (0.012) 0.085 (0.014) 0.108 (0.015) 0.243 (0.080) 0.261 (0.082) — 4.528 (0.102) β (SE) Race .242 *** * *** 0.670 *** 0.138 *** *** ** ** *** p 0.099 (0.016) −0.009 (0.012) (0.032) −0.135 0.085 (0.039) 0.070 (0.014) −0.0003 (0.013) 0.210 (.019) 0.018 (0.012) 0.086 (0.014) 0.119 (0.015) 0.172 (0.080) 0.171 (0.083) — 4.629 (0.100) β (SE) Politics (Continues) *** 0.417 *** * *** 0.979 *** 0.147 *** *** * * *** p LAW & POLICY Ni-WILEYJ_ 17 (Continued) 4159.055 2333 38 AIC BIC No. of counties No. of states 0.209 (0.457) 38 2333 3895.525 3849.486 3833.5 0.282 (0.531) Abbreviations: HLM, Hierarchical Linear Modeling; REML, restricted maximum likelihood methods. *p < .05; **p < .01; ***p < .001. 4120.3 4130.281 REML criterion 0.227 (0.476) 0.322 (0.568) Residual variance (SD) β (SE) β (SE) p Crime County type State intercept variance (SD) Random effects TABLE 2 p 38 2333 3795.706 3732.402 3710.4 0.267 (0.516) 0.160 (0.400) β (SE) Poverty p 38 2333 3805.375 3724.807 3696.8 0.263 (0.513) 0.158 (0.397) β (SE) Race p 38 2333 3781.327 3695.003 3665 0.260 (0.510) 0.137 (0.370) β (SE) Politics p 18 UNDERSTANDING THE PLACE OF PUNISHMENT LAW & POLICY Ni-WILEYJ_ 19 discretion (and the impact of local norms and dynamics on that discretion) in legal decisionmaking (Davis, 2008; Gottschalk, 2015; Lynch, 2011, 2016; Pfaff, 2017; Stuntz, 2011; Verma, 2016). The findings presented here indicate that change in the proportion of the population that identifies as non-Hispanic and White is not a significant predictor of prison admission rates. This may reflect the fact that while migrant settlement patterns have shifted, as scholars have noted, the non-Hispanic White population has actually declined more in the urban counties than in the nonurban counties included in our sample (see Table 1). These same urban counties are increasingly liberal and are, presumably, electing prosecutors and judges that reflect this political orientation. On the other hand, the size of the Black population is a significant predictor of prison admissions, though this relationship is nonlinear. That is, the size of the Black population matters more in rural and suburban counties than in urban counties with larger Black populations. This finding is consistent with prior studies that also find that the size of the Black population matters less in jurisdictions with very large Black populations, presumably because Black communities are able to secure a greater degree of political power where they comprise a comparatively large share of the population (Blalock, 1967; Keen & Jacobs, 2009). This finding is also consistent with the observation that prosecutors and other officials in large urban counties that are generally home to comparatively large Black populations appear to be embracing criminal justice reform more enthusiastically than their counterparts in less urban counties. Although the size of the Black population is a significant predictor of prison admissions, heightened use of prison admissions in (comparatively White) rural and suburban counties may be reducing levels of racial disproportionality in prison admissions observed during the height of mass incarceration. Prior research indicates that the impact of some of the correlations documented in the quantitative results may be bidirectional. That is, rising poverty and marginality in nonurban areas may fuel incarceration, and incarceration may, in turn, exacerbate poverty-related problems. This supposition is supported by evidence of mass incarceration’s adverse effects on justiceinvolved people, as well on as their families and their communities (Clear, 2007; Comfort, 2007; Lee et al., 2014, 2015; Sykes and Pettit, 2014; Wakefield & Wildeman, 2013; Wakefield et al., 2016; Western, 2006; Wildeman & Western, 2010). These adverse effects work to enhance poverty among the justice-involved and therefore to enhance inequality. For example, incarceration reduces earnings and employment, increases housing instability and indebtedness, and impairs the physical and mental health of the formerly incarcerated (Harris et al., 2010; Sewell & Jefferson, 2016; Travis et al., 2014; Western, 2006, 2012). High incarceration rates also impoverish, destabilize, and undermine the health and well-being of the families of people with conviction records (Comfort, 2007; Lee et al., 2014; Wakefield & Wildeman, 2013; Western, 2006; Wildeman & Western, 2010). The findings presented here thus have implications for sociological studies of mass incarceration, which often treat the carceral state as a means by which urban inequality is reproduced over time (Simes, 2018). To the extent that nonurban areas are addressed in this literature, they are primarily conceptualized as sites of prison construction and employment (e.g., Bonds, 2013; Eason, 2017) and, relatedly, as sources of political opposition to criminal justice reform (e.g., Thorpe, 2015). The possibility that the penal system plays a role in the lives of poor residents of nonurban communities that is akin to that observed in the nation’s largest cities has not been systematically addressed; research regarding the role of penal institutions in the lives of the rural and suburban poor would therefore fill an important lacuna. Similarly, a deeper understanding of the dynamics that are leading county officials in suburban and rural areas, especially those characterized by more conservative electorates, to rely extensively on prisons to cope with poverty-related problems is needed. In short, mass incarceration has long been conceptualized as a key component of urban inequality, but this is no longer appropriate (see also Kang-Brown et al., 2018; Simes, 2018). UNDERSTANDING THE PLACE OF PUNISHMENT 20 Instead, the changing spatial distribution of poverty-related problems and the intensification of political divisions that increasingly fall along the urban/rural continuum help to explain why prison admission rates are now higher in rural and suburban communities than in urban counties—despite the fact that the latter have notably higher crime rates. Given what is now known about how high incarceration rates fuel inequality (see especially Western, 2006), the findings presented here suggest the very real possibility that heightened penality is increasingly reproducing poverty and inequality not only in urban areas but also in rural and suburban communities across the country. This study also has important policy implications. Although discretion is ubiquitous and inevitable in the criminal legal process, studies show that the availability of “statutory hammers” such as mandatory minimum sentencing laws enable local criminal justice actors to impose harsh sentences where they are inclined to do so (Beckett & Beach, 2020; Lynch, 2011, 2016). By contrast, there is evidence that limiting statutory maximums effectively constrains the use of prisons. In Washington state, for example, the use of a determinate sentencing grid that mandates comparatively moderate sentences for drug and property offenses means that in many cases rural counties simply cannot send drug or property law violators to prison or for as long as they can in states that do not impose statutory maximums (Beckett & Beach, 2020). Thus, while state policies that expand the number and range of “statutory hammers” give toughminded prosecutors and judges (increasingly concentrated in nonurban locales) the tools they need to fill prisons and jails, sentencing reforms that reduce maximum sentence length could effectively constrain zealous prosecutors and judges located in otherwise punitive (and likely nonurban) counties. Given the human costs of mass incarceration and the increasingly central role it plays in the reproduction of poverty, policymakers across the country would be well advised to undertake such measures. A CK NO W L E D G M E N T S This research was supported by the National Science Foundation’s Law and Social Science program (#1456180). Thanks to E. Ann Carson at the Bureau of Justice Statistics for technical support. We are also grateful for feedback and suggestions from Heather Evans and Bruce Western. E N D N OT E S 1 Case and Deaton (2015) do not analyze morbidity and mortality rates by jurisdiction type. However, their findings reveal a sharp rise in morbidity and mortality rates among middle-aged non-Hispanic White men and women with lower levels of education. This population is disproportionately concentrated in rural areas. 2 Comparative studies suggest that race, politics, and racial politics are especially likely to affect penal outcomes in the United States, a decentralized federalist system in which elites are incentivized to attempt to shape and respond to public opinion and where the sentiments of electorally important segments of the public may have undue weight. By contrast, in more corporatist and centralized systems, decision-makers are less accountable and responsive to voters (Jacobs and Kleban, 2003; Savelsberg, 1994). 3 While studies focusing on the 1980s, 1990s, and 2000s consistently report such findings, several recent studies find that the strength of the Republican party at the state level does not predict incarceration rates after 2010 (Brown, 2016; Campbell et al., 2015). This shift could indicate that Democrats were able to position themselves as leaders in addressing public safety. Alternatively, the fact that the partisan divide now falls mainly along urban– rural rather than along state or regional lines might mean that the strength of the Republican Party now matters more on the county rather than on the state level. 4 Of the 2.2 million people incarcerated in the United States in 2014, 63.7% were housed in state prisons, while 26.7% and 9.6% were housed in local jails and federal prisons, respectively (Kaeble et al. 2015, Table 1). 5 In most states, people sentenced to less than one year of confinement serve their sentences in jail, while those sentenced to a year or more serve their time in prison. However, in a few states, people sentenced to less than one year of confinement serve their sentences in prison. To make the data comparable across states, we include only those sentenced to serve twelve or more months of prison confinement (see also Raphael & Stoll, 2013; Western, 2006). 6 Data available at https://www.cdc.gov/nchs/data/data_acces_files/NCHSURCodes2013.xlsx. LAW & POLICY Ni-WILEYJ_ 21 7 County-level UCR arrest data were unavailable for Florida and Illinois. For these states, arrest data from state police departments were used as a supplement. Additional information about the incorporation of these data is available in Appendix B. 8 According to the Bureau of Justice Statistics, only 0.7% of all state prisoners were incarcerated as a result of a simple marijuana possession conviction in 1997. Marijuana laws have loosened considerably since that time. 9 Data available at https://www.american.edu/spa/ccps/data-sets.cfm. 10 Data available at http://www.cnn.com/election/2012/results/state/AZ/president/. 11 Some prior research suggests that there is also regional variation in prison admission rates, with southern states having higher rates than the rest of the country. However, inclusion of controls for region would stress the normality assumption of the level-three error distribution and bias results (Maas & Hox, 2004), and thus a regional control was not included. R E FE RE NC E S Alexander, Michelle. 2010. The New Jim Crow: Mass Incarceration in the Age of Color Blindness. New York, NY: The New Press. Badger, Emily, Quoctrung Bui, and Adam Pearce. 2016. “The Election Highlighted a Growing Rural-Urban Split.” The New York Times, November 11, 2016. Beckett, Katherine. 1997. Making Crime Pay: Law and Order in Contemporary American Politics. New York, NY: Oxford University Press. Beckett, Katherine, and Lindsey Beach. 2020. “The Place of Punishment in 21st Century America: Understanding the Persistence of Mass Incarceration.” Law and Social Inquiry. 46(1): 1–31. https://doi.org/10.1017/lsi.2020.4 Beckett, Katherine, and Megan Ming Francis. 2021. In press. “The Origins of Mass Incarceration: The Racial Politics of Crime and Punishment in the Post-Civil Rights Era.” Annual Review of Law and Social Science. Beckett, Katherine, and Bruce Western. 2001. “Governing Social Marginality: Welfare, Incarceration, and the Transformation of State Policy.” In Mass Imprisonment: Its Causes and Consequences, edited by David Garland. Beverly Hills, CA: Sage Publications. Blalock, Hubert M. 1967. Toward a Theory of Minority Group Relations. New York, NY: Wiley. Blumstein, Alfred, and Allen J. Beck. 1999. “Population Growth in US Prisons, 1980–1996.” Crime and Justice 26: 17–61. Bonds, Anne. 2013. “Economic Development and Relational Racialization: ‘Yes in My Backyard’ Prison Politics and the Racialized Reinvention of Madras, Oregon.” Annals of the Association of American Geographers 103(6): 1389–405. Bridges, George S., Robert D. Crutchfield, and Edith E. Simpson. 1987. “Crime, Social Structure and Criminal Punishment: White and Nonwhite Rates of Imprisonment.” Social Problems 34(4): 345–61. Brown, Elizabeth K. 2016. “Toward Refining the Criminology of Mass Incarceration: Group-Based Trajectories of US States, 1977–2010.” Criminal Justice Review 2016: 1–19. Brown, Elizabeth K., and Kelly M. Socia. 2017. “Twenty-First Century Punitiveness: Social Sources of Punitive American Views Reconsidered.” Journal of Quantitative Criminology 33: 935–59. Burton, Linda M., Daniel T. Lichter, Regina S. Baker, and John M. Eason. 2013. “Inequality, Family Processes, and Health in the ‘New’ Rural America.” American Behavioral Scientist 57(8): 1128–51. Campbell, Michael C., and Heather Schoenfeld. 2013. “The Transformation of America’s Penal Order: A Historicized Political Sociology of Punishment.” American Journal of Sociology 118(5): 1375–423. Campbell, Michael C., Matt Vogel, and Joshua Williams. 2015. “Historical Contingencies and the Evolving Importance of Race, Violent Crime, and Region in Explaining Mass Incarceration in the United States.” Criminology 53: 180–203. Case, Anne, and Angus Deaton. 2015. “Rising Morbidity and Mortality in Midlife among White Non-Hispanic Americans in the 21st Century.” Proceedings of the National Academy of Science 112(49): 15078–83. Clear, Todd. 2007. Imprisoning Communities: How Mass Incarceration Makes Disadvantaged Communities Worse. Oxford: Oxford University Press. Clear, Todd R., and James Austin. 2009. “Reducing Mass Incarceration: Implications of the Iron Law of Prison Populations.” Harvard Law & Policy Review: 3: 307–24. Comfort, Megan. 2007. Doing Time Together: Love and Family in the Shadow of the Prison. Chicago, IL: University of Chicago Press. Davey, Joseph D. 1998. The Politics of Prison Expansion: Winning Elections by Waging War on Crime. Westport, CT: Praeger. Davis, Angela J. 2008. “The American Prosecutor: Power, Discretion, and Misconduct.” Criminal Justice 1: 24–37. Donato, Katharine M., Charles Tolbert, Alfred Nucci, and Yukio Kawano. 2008. “Changing Faces, Changing Places: The Emergence of New Nonmetropolitan Immigrant Gateways.” In New Faces in New Places: The Changing Geography of American Immigration, edited by Douglas S. Massey. New York, NY: Russell Sage. 22 UNDERSTANDING THE PLACE OF PUNISHMENT Eason, Jason. 2017. Big House on the Prairie. Chicago, IL: University of Chicago Press. Edsall, Thomas. 2017. “Reaching Out to the Voters Left Behind.” New York Times, April 13, 2017. Farrigan, Tracey. 2014. Poverty and Deep Poverty Increasing in Rural America. Washington, DC: US Department of Agriculture. Garland, David. 1990. Punishment and Modern Society: A Study in Social Theory. Chicago, IL: University of Chicago Press. Gottschalk, Marie. 2015. Caught: The Prison State and the Lockdown of American Politics. Princeton, NJ: Princeton University Press. Greenberg, David, and Valerie West. 2001. “State Prison Populations and Their Growth, 1971–1991.” Criminology 39: 615–54. Grusky, David B., Bruce Western, and Christopher Wimer, eds. 2011. The Great Recession. New York, NY: Russell Sage. Harris, Alexes, Heather Evans, and Katherine Beckett. 2010. “Drawing Blood from Stones: Legal Debt and Social Inequality in the Contemporary United States.” American Journal of Sociology 115(6): 1753–99. Helms, Ronald, and David Jacobs. 2002. “The Political Context of Sentencing: An Analysis of Community and Individual Determinants.” Social Forces 81(2): 577–604. Hinds, Oliver, Olive Lu, and Jacob Kang-Brown. 2018. Working Paper: Reconstructing How Counties Contribute to State Prison. New York, NY: Vera Institute of Justice. Housing Assistance Council. 2012. Race & Ethnicity in Rural America. Washington, DC: The Housing Assistance Council. Ingram, Deborah D., and Shelia J. Franco. 2014. 2013 NCHS Urban–Rural Classification Scheme for Counties. Centers for Disease Control and Prevention National Center for Health Statistics. Vital and Health Statistics Series 2, Number 166. Hyattsville, MD: US Department of Health and Human Services. Jacobs, David, and Jason T. Carmichael. 2001. “The Politics of Punishment across Time and Space: A Pooled Time Series Analysis of Imprisonment Rates.” Social Forces 80: 61–89. Jacobs, David, and Ronal Helms. 1999. “Collective Outbursts, Politics, and Punitive Resources: Toward a Political Sociology of Spending on Social Control.” Social Forces 77: 1497–524. Jacobs, David, and Richard Klebans. 2003. “Political Institutions, Minorities, and Punishment: A Pooled CrossNational Analysis of Imprisonment Rates.” Social Forces 82(2): 725–55. Jacobs, David, and Aubrey Jackson. 2010. “On the Politics of Imprisonment: A Review of Systematic Findings.” Annual Review of Law and Social Science 6: 129–49. Kaeble, Danielle, Lauren, Glaze, Anastasios Tsoutis, and Todd Minton. 2014. Correctional Populations in the United States. Washington D.C.: Bureau of Justice Statistics, 2015. Kang-Brown, Jacob, Oliver Hinds, Jasmine Heiss, and Olive Lu. 2018. The New Dynamics of Mass Incarceration. New York, NY: The Vera Institute for Justice. Keen, Bradley, and David Jacobs. 2009. “Racial Threat, Partisan Politics, and Racial Disparities in Prison Admissions: A Panel Analysis.” Criminology 47(1): 209–38. Keller, Josh, and Adam Pearce. 2016. “A Small Indiana County Sends More People to Prison Than San Francisco and Durham, N.C., Combined. Why?” New York Times, September 2, 2016. Keyes, Katherine M., Magdalena Cerda, Joanne E. Brady, Jennifer R. Havens, and Sandro Galea. 2014. “Understanding the Rural–Urban Differences in Nonmedical Prescription Opioid Use and Abuse in the United States.” American Journal of Public Health 104(2): e52–9. King, Ryan. 2007. “Group Threat and Social Control: Race, Perceptions of Minorities, and the Desire to Punish.” Social Forces 85(2): 1254–80. Kneebone, Elizabeth. 2017. The Changing Geography of US Poverty. Washington, DC: Brookings Institute. https:// www.brookings.edu/testimonies/the-changing-geography-of-us-poverty/ Kneebone, Elizabeth, and Alan Berube. 2013. Confronting Suburban Poverty in America. Washington, DC: Brookings Institution Press. Kohler-Hausman, Julilly. 2017. Getting Tough: Welfare and Imprisonment in 1970s America. Princeton, NJ: Princeton University Press. Kron, Josh. 2012. “Red State, Blue City: How the Urban–Rural Divide is Splitting America.” The Atlantic 30, 2012. https://www.theatlantic.com/politics/archive/2012/11/red-state-blue-city-how-the-urban-rural-divide-is-splittingamerica/265686/. Lacy, Karyn. 2016. “The New Sociology of Suburbs: A Research Agenda for Analysis of Emerging Trends.” Annual Review of Sociology 42: 369–84. Lee, Hedwig, Tyler McCormick, Margaret T. Hicken, and Christopher Wildeman. 2015. “Racial Inequalities in Connectedness to Imprisoned Individuals in the United States.” Du Bois Review 12(2): 269–82. Lee, Hedwig, Christopher Wildeman, Emily Wang, Niki Matusko, and James S. Jackson. 2014. “A Heavy Burden? The Health Consequences of Having a Family Member Incarcerated.” American Journal of Public Health 104(3): 421–7. LAW & POLICY Ni-WILEYJ_ 23 Lichter, Daniel T., Domenico Parisi, and Michael C. Tacquino. 2012. “The Geography of Exclusion: Race, Segregation, and Concentrated Poverty.” Social Problems 59: 364–88. Liska, Allen E., Joseph J. Lawrence, and Andrew Sanchirico. 1982. “Fear of Crime as a Social Fact.” Social Forces 60 (3): 760–70. Love, Michael M., Tracy J. Cohn, Thomas W. Pierce, and Sarah L. Hastings. 2016. “Trends in Injection Use among Prescription Opioid-Misusing Individuals in the Rural United States.” Journal of Rural Mental Health 40(3): 180–92. Lynch, Mona. 2011. “Mass Incarceration, Legal Change, and Locale: Understanding and Remediating American Penal Overindulgence.” Criminology and Public Policy 10(3): 273–98. Lynch, Mona. 2016. Hard Bargains: The Coercive Power of Drug Laws in Federal Court. New York, NY: Russell Sage Foundation. Maas, Cora J. M., and Joop J. Hox. 2004. “Robustness Issues in Multilevel Regression Analysis.” Statistica Neerlandica 58(2): 127–37. Massey, Douglas S., and Chiara Capoferro. 2008. “The Geographic Diversification of American Immigration.” In New Faces in New Places: The Changing Geography of American Immigration, edited by Douglas S. Massey. New York, NY: Russell Sage. Mather, Mark, and Beth Jarosz. 2016. Poverty and Inequality Pervasive in Two-Fifths of US Counties. Washington, DC: Population Reference Bureau. https://www.prb.org/poverty-and-inequality-us-counties/ Monnat, Shannon M. 2018. “Factors Associated with County-Level Differences in US Drug-Related Mortality Rates.” American Journal of Preventative Medicine 54(5): 611–9. Myers, Sage R., Charles C. Branas, Benjamin C. French, Michael L. Nance, Michael J. Kallan, Douglas J. Wiebe, and Brendan G. Carr. 2013. “Safety in Numbers: Are Major Cities the Safest Places in the United States?” Annals of Emergency Medicine 62(4): 408–18. Neal, Derek, and Armin Rick. 2014. “The Prison Boom and the Lack of Black Progress after Smith and Welch.” National Bureau of Economic Research, Working Paper No. 20283, 2014. Pfaff, John F. 2017. Locked In: The True Causes of Mass Incarceration and How to Achieve Real Reform. New York, NY: Basic Books. Quinones, Sam. 2015. Dreamland: The True Tale of America’s Opiate Epidemic. New York, NY: Bloomsbury Press. Raphael, Steven, and Michael A. Stoll. 2010. Job Sprawl and the Suburbanization of Poverty. Washington, DC: Brookings Institution. Raphael, Steven, and Michael A. Stoll. 2013. Why Are So Many Americans in Prison?. New York, NY: Russell Sage Foundation. Reding, Nick. 2009. Methland: The Death and Life of an American Small Town. New York, NY: Bloomsbury Press. Rodgers, Simon, and Feilding Cage. 2012. “Full US 2012 Election County-Level Results to Download.” The Guardian. https://www.theguardian.com/news/datablog/2012/nov/07/us-2012-election-county-results-download. Raudenbush, Stephen W., and Anthony S. Bryk. 2002. Hierarchical Linear Models: Applications and Data Analysis Methods. Beverly Hills, CA: Sage Publications. Sampson, Robert J. 2012. Great American City: Chicago and the Enduring Neighborhood Effect. Chicago, IL: University of Chicago Press. Sampson, Robert J. 2014. “Criminal Justice Processing and the Social Matrix of Adversity.” Annals of the Academy of Social and Political Sciences 651: 296–301. Sampson, Robert, and Charles Loeffler. 2010. “Punishment’s Place: The Local Concentration of Mass Incarceration.” Daedalus: 139(3): 20–31. Savelsberg, Joachim J. 1994. “Knowledge, Domination, and Criminal Punishment.” American Journal of Sociology 99: 911–43. Sewell, Abigail, and Kevin Jefferson. 2016. “Collateral Damage: The Health Effects of Invasive Police Encounters in New York City.” Journal of Urban Health 93(Supplement 1): 42–67. Simes, Jessica. 2018. “Place and Punishment: The Spatial Context of Mass Incarceration.” Journal of Quantitative Criminology March 24 online. 34(2): 513–33. Singer, Audrey. 2011. Immigrants in 2010 Metropolitan Areas: A Decade of Change. Washington, DC: Brookings Institution. Soss, Joe, Richard C. Fording, and Sanford F. Schram. 2011. Disciplining the Poor: Neoliberal Paternalism and the Persistent Power of Race. Chicago, IL: University of Chicago Press. Stuntz, William J. 2011. The Collapse of American Criminal Justice. Cambridge: Harvard University Press. Subramanian, Ram, Christian Henrichson, and Jacob Kang-Brown. 2015. In Our Own Backyard: Confronting Growth and Disparities in Local Jails. New York, NY: Vera Institute. Sykes, Bryan, and Becky Pettit. 2014. “Mass Incarceration, Family Complexity, and the Reproduction of Childhood Disadvantage.” Annals of the American Academy of Political and Social Sciences, 654(1): 127–49. Thorpe, Rebecca. 2015. “Perverse Politics: The Persistence of Mass Imprisonment in the 21st Century.” Perspectives on Politics 13(3): 618–37. UNDERSTANDING THE PLACE OF PUNISHMENT 24 Travis, Jeremy, Bruce Western, and Stephan Redburn, eds. 2014. The Growth of Incarceration in the United States: Exploring Causes and Consequences. Washington, DC: The National Academies Press. Truman, Jennifer L., and Lynn Langton. 2015. Criminal Victimization, 2014. Washington, DC: Department of Justice. Tyler, Tom R., and Robert J. Boekmann. 1997. “Three Strikes and You’re Out—But Why? The Psychology of Public Support for Punishing Rule Breakers.” Law & Society Review 31(2): 237–65. Venkatesh, Sudhir, and Steven D. Levitt. 2000. “An Economic Analysis of a Drug-Selling Gang’s Finances..” The Quarterly Journal of Economics 115(3): 755–89. Verma, Anjuli. 2016. “The Law-Before: Legacies and Gaps in Penal Reform.” Law and Society Review 49(4): 847–82. Wakefield, Sara, Hedwig Lee, and Christopher Wildeman. 2016. “Tough on Crime, Tough on Families? Criminal Justice and Family Life in America.” Annals of the American Academy of Political and Social Science 665: 8–21. Wakefield, Sara, and Christopher Wildeman. 2013. Children of the Prison Boom: Mass Incarceration and the Future of American Inequality. Oxford: Oxford University Press. Weaver, Vesla. 2007. “Frontlash: Race and the Development of Punitive Crime Policy.” Studies in American Political Development 21: 230–65. Weidner, Robert R., and Richard S. Frase. 2003. “Legal and Extralegal Determinants of Intercounty Differences in Prison Use.” Criminal Justice Policy Review 14(3): 377–400. Wells, L. Edward, and Ralph A. Weisheit. 2004. “Patterns of Rural and Urban Crime: A County-Level Comparison.” Criminal Justice Review 29(1): 1–22. Western, Bruce. 2006. Punishment and Inequality. New York, NY: Russell Sage Foundation. Western, Bruce. 2012. “The Impact of Incarceration on Wage Mobility and Inequality.” American Sociological Review 67: 526–46. Wildeman, Christopher, and Bruce Western. 2010. “Incarceration in Fragile Families.” The Future of Children 20(2): 157–77. AUTHOR BIOGRAPHIES Katherine Beckett is Chair and Professor in the Department of Law, Societies, and Justice and S. Frank Miyamoto Professor of Sociology at the University of Washington in Seattle. Lindsey Beach is a PhD student in the Department of Sociology at the University of Washington, where she studies topics related to social control, mental health, and criminal punishment. ============ I How to cite this article: Beckett K, Beach L. Understanding the place of punishment: Disadvantage, politics, and the geography of imprisonment in 21st century America. Law & Policy. 2021;5–29. https://doi.org/10.1111/lapo.12161 A PPE ND I X A : FACTOR LOADINGS FOR CONCENTRATED DISADVANTAGE INDEX Variable Factor loading Percent poverty (2012) 0.503 Percent female-headed household (2012) 0.467 Percent unemployed (2012) 0.324 Percent less than a high school education (2012) 0.462 Age-adjusted premature death rate (2008–2012) 0.459 I LAW & POLICY Ni-WILEYJ_ 25 A PPE ND I X B : ADDITIONAL INFORMATION REGARDING DATA SOURCES AND MEASUREMENT Our HLM samples consist of 2333 counties from 38 states across the United States. Inclusion in the sample was determined by the availability of prison admission, offense, and arrest data. Thirty-three states (of our thirty-eight-state sample) reported sufficient NCRP term records data. For Hawaii, Iowa, and Minnesota, we also use NCRP data, but the data specifications for these states vary slightly. Hawaii’s NCRP data are taken from Part A (admissions) data, rather than term records data. Minnesota’s data are from the NCRP term records, but do not include sentence length. We therefore compared NCRP admission figures with bureau of justice statistics admission data (restricted to greater than twelve months) and confirmed that these were very similar. Admissions data for Kansas and Mississippi were taken from state department of corrections reports. Inclusion also depended upon the quality of UCR data. Comparisons between offense and arrest populations indicate whether the UCR data can be used to create reliable arrest-tooffense ratios; inclusion of a similar population size for both data sources was critical to ensure that this measure was not skewed due to uneven reporting. Counties with large (i.e., greater than 10%) discrepancies between the populations sampled for the crime and arrest data were dropped, along with counties that had fully imputed crime and arrest data. A total of 246 counties were dropped from the sample for this reason. Florida and Illinois did not report UCR arrest data at the county level. However, both states report these data through their respective state police departments, and these data were included for both time periods. In addition, while Florida does not report detailed drug arrest data, it does include information regarding total drug arrests. To calculate a comparable felony drug arrest for these two states, county-level data were multiplied by 0.495 (because 49.5% of arrests for drug abuse violations were not for marijuana possession, an arrest that rarely leads to a felony conviction). California was omitted from the sample because of the impact of the Brown v Plata (2011) ruling. In response to this court decision, California began shrinking its prison population through early release and holding inmates with sentences of up to four years in county jails rather than state prisons (Verma, 2016). Including California NCRP prison admission data, which omits many people serving confinement sentences of more than one year, would therefore have artificially biased the results. Our dependent variable is the logged average prison admission rate in 2013. These data include admissions for all new commitments with sentences longer than twelve months stemming from either new convictions or probation violations. The reliability of NCRP admission data were assessed by comparing state totals for each year with the Bureau of Justice Statistics’ Prisoners in the US report, which details total admissions (for sentences greater than twelve months) for each state. Additional reliability information was also gleaned from the Vera Institute of Justice’s Working Paper: Reconstructing How Counties Contribute to State Prisons (Hinds et al., 2018). 1. Drug-related death rate: Drug poisoning death data come from the CDC’s Multiple Cause of Death Files. This measure captures the number of drug poisoning deaths (for all individuals) divided by the total county population from 2008 to 2012. Longer time periods were included for this measure because the CDC suppresses values smaller than 10 for anonymity reasons; by including data for a greater number of years in the measure, we were able to reduce the number of counties with missing data. Drug poisonings include any deaths with at least one cause of death from the following: accidental drug poisoning (MCD ICD-10 codes X40–X44), intentional self-poisoning by drugs (codes X60–X64), assault by drugs (code X85), and poisoning by and exposure to drugs not elsewhere classified (i.e., with an UNDERSTANDING THE PLACE OF PUNISHMENT 26 undetermined intent—codes Y10–Y14). Rates are measured per 100,000 individuals. A total of 786 counties had suppressed data (33.6% of the total sample). These missing values were replaced using multiple imputation methods. 2. Opioid prescribing rate: Opioid prescribing rates for 2013 measure the number of prescriptions per 100 residents and are included as a proxy measure of the opioid crisis on the county level. These data were retrieved from the CDC (data available at https://www.cdc.gov/ drugoverdose/maps/rxcounty2012.html), which compiled the data from the QuintilesIMS Transactional Data Warehouse. QuintilesIMS gathers data on opioid prescriptions in the U. S. from a sample of nonhospital retail pharmacies. These pharmacies dispense approximately 88% of all opioid prescriptions in the United States. Values are missing for 237 counties (10.1% of the total sample) and were replaced using multiple imputation techniques. The construction of many independent variables is self-evident based on their description. Further information regarding the premature age-adjusted mortality rate, drug-poisoning death rate, and opioid prescribing rate are provided below. • Premature age-adjusted mortality rate: This measure was calculated by dividing the total number of deaths of people under seventy-five years of age by the county population. This measure is age-adjusted by the standardized 2000 U.S. population and is the average rate from 2008 through 2012. Due to CDC procedures that suppress data when total death counts are less than ten for a geographic area, fifteen counties are missing data for this variable (less than 1% of the total sample). These data were replaced using multiple imputation techniques. A PPE ND I X C : SAMPLED STATES AND COUNTIES State Region Total number of counties Sampled counties Alabama South 67 60 Arizona West 15 15 Colorado West 64 55 Florida South 67 66 Georgia South 159 148 Hawaii West 5 4 Illinois Midwest 102 101 Indiana Midwest 92 66 Iowa Midwest 99 90 Kansas Midwest 105 83 Kentucky South 120 113 Maine Northeast 16 16 Maryland South 24 24 Massachusetts Northeast 14 14 Michigan Midwest 83 79 Minnesota Midwest 87 84 Mississippi South Missouri Midwest 82 44 114 114 (Continues) LAW & POLICY Ni-WILEYJ_ State Region Nebraska Midwest 93 58 Nevada West 17 16 New Jersey Northeast 21 21 New York Northeast North Carolina South North Dakota Total number of counties Sampled counties 62 62 100 90 Midwest 53 43 Ohio Midwest 88 77 Oklahoma South 77 72 Oregon West 36 34 Pennsylvania Northeast 67 67 Rhode Island Northeast 5 4 South Carolina South 46 45 South Dakota Midwest 66 36 Tennessee South 95 95 Texas South 254 242 Utah West 29 24 Washington West 39 39 West Virginia South 55 41 Wisconsin Midwest 72 70 Wyoming West Sample total 27 23 21 2612 2333 0.338 (0.081) 0.947 0.590 −0.005 (0.083) −0.045 (0.083) Rural Suburban 0.172 (.014) 0.108 (0.014) 0.045 (0.013) — — — — — — — — — — — — — — — Felony Offense Rate Drug arrest rate (logged) Arrest to offense ratio Concentrated disadvantage Drug death rate (logged) Opioid prescribing rate % Black % Black (squared) Change in % White 0.236 (0.080) — — County type (Urban) 4.954 (0.102) 5.523 (0.106) *** β (SE) p β (SE) (Intercept) Fixed effects Crime County type *** *** *** ** *** *** p — — — 0.092 (0.014) 0.005 (0.013) 0.147 (0.017) 0.020 (0.012) 0.092 (0.015) 0.110 (0.015) 0.227 (0.079) 0.230 (0.080) — 4.948 (0.100) β (SE) Poverty *** 0.644 *** 0.091 *** *** ** ** *** p −0.012 (0.032) −0.139 0.084 (0.039) 0.08 (0.014) 0.000 (0.013) 0.181 (0.019) 0.018 (0.012) 0.085 (0.014) 0.111 (0.015) 0.237 (0.080) 0.253 (0.082) — 4.905 (0.101) β (SE) Race APP EN D IX D: OLS RESULTS: COUNTY-LEVEL DETERMINANTS OF PRISON ADMISSIONS, 2013 0.290 *** *** *** 0.752 *** 0.149 *** *** * * *** p −0.008 (0.032) −0.134 −0.060 (0.019) 0.074 (0.014) −0.007 (0.013) 0.202 (0.019) 0.018 (0.012) 0.090 (0.014) 0.090 (0.014) 0.127 (0.015) 0.104 (0.082) — 4.954 (0.100) β (SE) Politics (Continues) 0.472 *** ** *** 0.595 *** 0.113 *** *** 0.136 0.206 *** p 28 UNDERSTANDING THE PLACE OF PUNISHMENT 2333 38 No. of counties No. of states Abbreviation: OLS, ordinary least squares. *p < .05; **p < .01; ***p < .001. 4019 4252 0.567 Residual SE AIC 0.356 Adjusted R2 BIC — % Romney vote 38 2333 3968 3968 0.531 0.435 — β (SE) β (SE) p Crime County type p 38 2333 3855 3855 0.516 467 — β (SE) Poverty p 38 2333 3857 3857 0.515 0.469 — β (SE) (0.012) Race p 38 2333 3830 3830 0.511 0.477 0.093 (0.016) β (SE) (0.012) Politics *** p LAW & POLICY Ni-WILEYJ_ 29